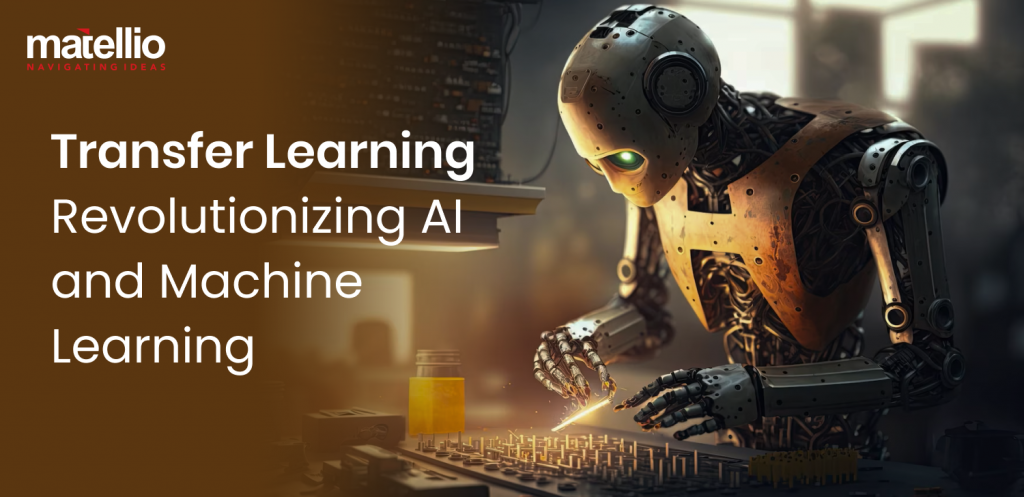
In the ever-evolving realm of AI and machine learning, transfer learning has emerged as a game-changer. This innovative technique involves repurposing knowledge acquired from solving one problem to tackle another, yet related, problem. Such an approach significantly accelerates the development of AI models and broadens their applicability across various domains.
Statistical Insights
The impact of transfer learning can be quantified by several compelling statistics.
1. Accelerated Development
According to a study by MIT, transfer learning can reduce the time required for model development by up to 40%. This is because it eliminates the need for building models from scratch for each new problem (MIT News).
2. Enhanced Efficiency
Research indicates that transfer learning can improve model accuracy by up to 15-20% in tasks like image classification and natural language processing, especially when the available training data for the new task is limited (ScienceDirect).
3. Cost-Effectiveness
A report by Deloitte highlights that businesses utilizing transfer learning can achieve a reduction in AI development costs by up to 30%, making AI solutions more accessible and scalable (Deloitte Insights).
4. Expanding Horizons in AI
Transfer learning not only streamlines the AI development process but also democratizes AI by making it more accessible to companies with limited data or technical resources. As a leading AI development company, the integration of transfer learning into AI strategies is a key focus. This approach opens doors to a wide range of applications, from enhancing healthcare diagnostic systems to refining financial fraud detection mechanisms.
The Role of AI Development Companies
AI development companies play a pivotal role in leveraging transfer learning to create innovative solutions. These companies specialize in identifying suitable pre-trained models and customizing them to meet specific business needs. They provide expertise in handling various aspects of transfer learning, including data adaptation, model tuning, and continuous improvement, thereby ensuring that AI models remain effective and relevant in rapidly changing business landscapes.
Benefits of Transfer Learning in AI and Machine Learning
1. Accelerated AI Model Development
Transfer learning significantly speeds up the development of AI models. By using pre-trained models, developers can bypass the time-consuming and resource-intensive phase of training models from scratch. This leads to quicker deployment of AI solutions.
2. Enhanced Model Performance
Models developed using transfer learning often demonstrate enhanced performance, especially in tasks like image and speech recognition. The pre-trained models have a foundational understanding of general patterns, which, when fine-tuned, can accurately adapt to specific tasks.
3. Cost-Effective AI Solutions
The approach is more cost-effective compared to traditional model training methods. By leveraging existing models, businesses can reduce the computational resources and time required for training, leading to lower development costs.
4. Accessibility to Small and Medium Enterprises
Transfer learning democratizes AI, making advanced AI technologies accessible to smaller businesses that may lack the resources for extensive model training. This opens up opportunities for innovation and competition in various sectors.
5. Versatility Across Multiple Domains
One of the key strengths of transfer learning is its versatility. The same foundational models can be adapted for diverse applications across different industries, such as healthcare diagnostics, financial fraud detection, and customer service enhancements.
6. Improved Data Efficiency
Transfer learning is particularly valuable in scenarios with limited data. Pre-trained models require less data to adapt to a new task, making it possible to develop effective AI models even with smaller datasets.
7. Reduced Risk of Overfitting
Since transfer learning models are pre-trained on large and diverse datasets, they are generally less prone to overfitting when compared to models trained from scratch on limited data.
8. Continuous Learning and Adaptation
Transfer learning models can continuously adapt and learn from new data, ensuring that AI solutions remain relevant and effective over time as they encounter new information and scenarios.
Transfer learning stands as a transformative approach in AI and machine learning, offering numerous benefits that streamline development, enhance performance, and widen accessibility. These advantages position transfer learning as a key strategy in modern AI development, fostering innovation and efficiency.
Transfer Learning Use Cases
Transfer Learning Use Case 1: Image Recognition
Image recognition, a critical application in AI, has been revolutionized by transfer learning. In medical imaging, for instance, transfer learning enables the development of highly accurate diagnostic models.
Here, models pre-trained on extensive image datasets are fine-tuned to detect specific conditions like tumors or fractures. This approach drastically reduces the need for large, specialized medical imaging datasets, which are often difficult to acquire due to privacy concerns and variability in medical cases.
Similarly, in the automotive industry, transfer learning propels the advancement of autonomous vehicle technologies. Vehicles equipped with AI can utilize transfer learning to better interpret and react to their surroundings.
For example, a model initially trained on a vast dataset of road images can be further refined to recognize specific traffic signs or pedestrian behaviors relevant to different geographical locations. This not only enhances the safety features of autonomous vehicles but also accelerates the development process by leveraging pre-existing data and knowledge.
The application of transfer learning in image recognition demonstrates its capacity to not only improve model accuracy but also to significantly shorten the development cycle by utilizing pre-trained models. This makes it an invaluable tool in fields where data is either scarce or requires significant resources to collect and label.
Transfer Learning Use Case 2: Natural Language Processing (NLP)
Natural Language Processing (NLP) has experienced a paradigm shift with the adoption of transfer learning. Large language models like GPT-3 have been pre-trained on vast amounts of text data, encompassing a wide range of topics and styles. This pre-training enables these models to have a fundamental understanding of language and context, which can then be fine-tuned for specific NLP tasks.
One prominent application is in creating more responsive and accurate chatbots. By utilizing a transfer learning approach, chatbots can be quickly adapted to understand and respond appropriately in various contexts, such as customer service, personal assistants, or therapy bots. This adaptability is crucial for businesses aiming to provide more personalized and effective customer interactions.
Another significant application is in sentiment analysis. Conversational AI service providers utilize transfer learning to fine-tune models for specific types of text, like social media posts or product reviews, enabling them to gain deeper insights into customer opinions and trends. This approach is particularly beneficial for marketing and product development strategies, as it allows companies to understand customer needs and preferences more accurately and rapidly.
The flexibility of transfer learning in NLP shows its ability to not only enhance the performance of language models but also to tailor them to diverse and specific requirements, making it a cornerstone technique in modern NLP applications.
Transfer Learning Use Case 3: Sentiment Analysis
Sentiment analysis, a key tool in understanding customer feedback, has been significantly enhanced by transfer learning. Traditionally, sentiment analysis models were limited by the scope of their training data, often struggling with nuances and context-specific interpretations. Transfer learning has changed this landscape.
By utilizing models initially trained on extensive and diverse text data, businesses can fine-tune these models to their specific domain, such as social media, product reviews, or customer support interactions.
This fine-tuning allows the models to grasp the subtleties and variations in language used across different platforms and demographics. For instance, the way customers express satisfaction or frustration can vary greatly between Twitter and formal product reviews. Transfer learning enables models to adapt to these differences, providing businesses with more accurate and nuanced insights into customer sentiments.
The impact of this is profound for marketing strategies, product development, and customer relationship management. Companies can quickly adapt their approaches based on real-time sentiment analysis, aligning their products and services more closely with customer expectations and needs. This responsiveness is crucial in today’s fast-paced market environment, where customer preferences and trends are constantly evolving.
Transfer Learning Use Case 4: Fraud Detection
In the financial sector, fraud detection is a critical application where transfer learning has made significant strides. Financial institutions are inundated with vast amounts of transaction data, making it challenging to identify fraudulent activities effectively. Traditional methods often require extensive feature engineering and domain-specific data preparation. Transfer learning, however, offers a more dynamic solution.
By leveraging models pre-trained on large-scale financial datasets, banks and other institutions can fine-tune these models to their specific transaction patterns and customer behaviors. This approach enhances the model’s ability to detect unusual and potentially fraudulent transactions more accurately. For instance, a model trained on international banking transactions can be adapted to focus on anomalies in regional transactions, accounting for local spending habits and trends.
The effectiveness of transfer learning in fraud detection is not just in its accuracy but also in its efficiency. It significantly reduces the time and resources needed to develop robust fraud detection systems. This is especially beneficial for smaller institutions that may lack the extensive datasets needed to train models from scratch. With transfer learning, they can implement state-of-the-art fraud detection systems, leveling the playing field in financial security.
Transfer Learning Use Case 5: Predictive Maintenance
Predictive maintenance is a vital application in industries such as manufacturing and aviation, where equipment failure can lead to significant downtime and costs. Transfer learning has emerged as a powerful tool in this domain. By leveraging models pre-trained on large datasets of machine operations and failures, companies can fine-tune these models to predict failures in their specific machinery.
This application of transfer learning is not only about predicting when a machine might fail but also about understanding the underlying causes of potential failures. This insight enables companies to perform maintenance activities proactively, reducing unplanned downtime and extending the life of their equipment. For industries where equipment maintenance represents a significant operational cost, this approach can lead to substantial savings and efficiency improvements.
The integration of transfer learning in predictive maintenance is a prime example of how digital transformation services are evolving. By adopting advanced AI techniques like transfer learning, companies can transform their traditional maintenance processes, leading to more proactive and data-driven decision-making.
Transfer Learning Use Case 6: Drug Discovery
In the pharmaceutical industry, drug discovery is a complex and time-consuming process. Transfer learning has the potential to significantly accelerate this process. By applying models initially trained on extensive biochemical and pharmacological data, researchers can adapt these models to identify potential drug candidates for specific diseases more efficiently.
This approach is particularly beneficial in the early stages of drug discovery, where identifying compounds that could potentially treat specific conditions is a major challenge. Transfer learning enables researchers to leverage existing knowledge and data, reducing the need for extensive and costly experimentation.
The application of transfer learning in drug discovery is a testament to the advancements in technology consulting services. Best bet when planning implementation is to Hire Chatbot Developers specializing in AI and machine learning as they can guide pharmaceutical companies in effectively implementing transfer learning techniques, leading to faster and more cost-effective drug development processes.
Transfer Learning Use Case 7: Retail Personalization
Retail personalization, crucial for enhancing customer experience and sales, has been transformed by transfer learning. In this domain, models pre-trained on extensive consumer behavior data are adapted to individual retailers’ specific customer demographics and preferences. This adaptation allows for more accurate product recommendations, tailored marketing strategies, and personalized customer interactions.
For example, an e-commerce platform can use transfer learning to fine-tune recommendation algorithms based on its unique product range and customer interactions. This leads to more relevant and engaging shopping experiences, driving both customer satisfaction and sales. The ability of transfer learning to quickly adapt to different retail environments demonstrates its versatility and power in personalizing customer experiences at scale.
This application aligns perfectly with the concept of custom machine learning solutions, where AI models are tailored to meet the specific needs of a business. Retailers, by leveraging custom ML solutions, can effectively implement transfer learning to enhance their customer engagement strategies, thereby aligning with their unique business goals and customer expectations.
Transfer Learning Use Case 8: Market Forecasting
Market forecasting in finance and economics is another area where transfer learning has shown significant impact. By applying models trained on vast datasets of market behavior and economic indicators, financial institutions and analysts can fine-tune these models to predict specific market trends or stock performances.
This approach enables more accurate and timely predictions, crucial for investment decisions and economic planning. For instance, a model trained on global financial data can be adapted to focus on emerging market trends, providing investors with insights that might not be evident from traditional analysis methods.
The integration of transfer learning in market forecasting is a significant step forward in the financial sector, offering a more data-driven and predictive approach to understanding market dynamics. This is particularly relevant in today’s volatile and fast-changing economic environment, where traditional forecasting methods may fall short.
Transfer Learning Use Case 9: Voice Assistants
Voice assistants, such as Siri and Alexa, have significantly improved through transfer learning. By utilizing large language models pre-trained on diverse datasets of human speech, these assistants can better understand and process various languages, accents, and speech patterns. The models are then fine-tuned to handle specific user interactions, making them more responsive and accurate.
This application is particularly important as it ensures inclusivity and accessibility in voice recognition technology. For instance, voice assistants can be adapted to understand regional accents or dialects, making technology more accessible to a broader range of users. This ability to rapidly adapt and learn from new voice data is crucial in creating user-friendly and efficient voice-activated systems.
Transfer Learning Use Case 10: Autonomous Vehicles
In the automotive industry, autonomous vehicles utilize transfer learning for improved safety and navigation. Models initially trained on extensive datasets of traffic situations, road conditions, and driver behaviors are adapted to specific environments or scenarios. This training allows autonomous vehicles to make more accurate real-time decisions, enhancing safety and efficiency.
For example, a model trained on data from urban driving scenarios can be fine-tuned for suburban or rural areas, accounting for differences in traffic density, road layouts, and pedestrian behaviors. This adaptability is key to the widespread adoption and reliability of autonomous driving technologies.
Read More: Discover how AI in rural healthcare is revolutionizing access to quality medical services and bridging the healthcare gap.
Transfer Learning Use Case 11: Agricultural Optimization
Agricultural optimization through transfer learning involves adapting models to improve crop yield predictions, pest detection, and soil health monitoring. Models trained on extensive datasets encompassing various crop types, weather conditions, and soil compositions are fine-tuned for specific agricultural environments.
This application enables farmers to make more informed decisions about planting, irrigation, and pest control, leading to increased efficiency and yield. For instance, a model trained on satellite and drone imagery can be adapted to monitor specific crop health, helping farmers detect and address issues like nutrient deficiencies or disease outbreaks early.
Transfer Learning Use Case 12: Weather Forecasting
Transfer learning has significantly advanced weather forecasting. Meteorologists now use models pre-trained on vast historical climate data and fine-tune them with current weather patterns to predict future weather conditions more accurately.
This approach is crucial for early warning systems and disaster preparedness, as it enhances the ability to predict extreme weather events like hurricanes, floods, or droughts. The improved accuracy and timeliness of these predictions can save lives and resources by allowing for better preparation and response to weather-related emergencies.
Transfer Learning Use Case 13: Language Translation Services
Language translation services have greatly benefited from transfer learning. By utilizing models like BERT, which are trained on multiple languages, companies can fine-tune these models for specific language pairs.
This results in translations that are not only more accurate but also contextually relevant, a critical factor in fields like legal or medical translations. Transfer learning has enabled these services to provide faster and more reliable translations, breaking down language barriers in global communication and business.
Transfer Learning Use Case 14: Facial Recognition
Facial recognition technology, widely used in security and surveillance, has been enhanced through transfer learning. By adapting models trained on diverse datasets of faces, this technology can now more accurately recognize individuals across various lighting conditions, angles, and facial expressions.
This advancement is significant for public safety, identity verification processes, and even for enhancing user experiences in consumer electronics.
Transfer Learning Use Case 15: Content Recommendation Systems
Content recommendation systems in streaming services like Netflix and Spotify have been refined using transfer learning. These systems analyze vast user data to understand preferences and viewing habits.
By employing transfer learning, these platforms can quickly adapt their algorithms to provide personalized content recommendations, significantly enhancing user engagement and satisfaction.
Transfer Learning Use Case 16: Healthcare Analytics
Healthcare analytics has been revolutionized by transfer learning. With models pre-trained on extensive health datasets, healthcare providers can fine-tune these models for specific medical conditions or patient demographics. This application is crucial in diagnosing diseases more accurately and developing personalized treatment plans.
For instance, a model trained on general health records can be adapted to predict the onset of chronic diseases like diabetes or heart conditions in specific populations, aiding in early intervention and better patient outcomes. The ability to customize models for particular healthcare scenarios significantly enhances patient care and operational efficiency in medical facilities.
Transfer Learning Use Case 17: Human Resource Management
Human Resource Management has seen a substantial impact from transfer learning. By utilizing models trained on vast datasets of employee performance, HR departments can adapt these models to predict employee success, identify potential leaders, or even anticipate turnover. This approach is particularly useful in large organizations with diverse workforces, where understanding and managing employee dynamics can be challenging.
For example, a model trained on general workforce data can be adapted to an organization’s specific cultural and operational context, helping HR teams to make more informed decisions about talent acquisition, training, and retention strategies.
Transfer Learning Use Case 18: Supply Chain Management
In supply chain management, transfer learning helps in improving demand forecasting and logistics planning. By applying models trained on broad logistics data, companies can fine-tune these for their specific supply chain challenges, such as route optimization or inventory management.
For example, a model initially trained on international supply chain data can be adapted to regional supply chain networks, allowing for more accurate predictions of delivery times and inventory needs. This tailored approach leads to more efficient and cost-effective supply chain operations, a crucial factor in today’s competitive business environment.
Transfer Learning Use Case 19: Energy Consumption Analysis
Transfer learning plays a significant role in energy management, particularly in analyzing and optimizing energy consumption. By leveraging models pre-trained on large-scale energy usage data, businesses can fine-tune these to their specific operational needs. This application enables more efficient use of energy, leading to cost savings and reduced environmental impact. For instance, a model trained on general energy consumption patterns can be adapted to predict and optimize energy use in specific types of buildings or industrial settings, contributing to more sustainable and efficient energy practices.
Transfer Learning Use Case 20: Educational Tools
Transfer learning has significantly influenced the development of educational tools, particularly in creating personalized learning experiences. Educational platforms can use models pre-trained on extensive datasets of student learning patterns and outcomes, and then fine-tune these models for specific educational content or student groups. This approach allows for the creation of adaptive learning systems that cater to individual student needs, optimizing learning paths based on their strengths and areas for improvement.
For example, a model trained on general student performance data can be adapted to identify the most effective teaching methods for different subjects or to provide customized support for students with specific learning challenges. This level of personalization in educational tools can lead to improved student engagement, better learning outcomes, and more efficient educational processes. The application of transfer learning in education is a key factor in advancing modern educational methodologies, making learning more accessible and effective for diverse student populations.
With these 20 use cases, the vast potential and versatility of transfer learning across various sectors and applications are evident. From enhancing medical diagnostics to optimizing supply chain management, transfer learning stands as a pivotal technology in the AI and machine learning landscape, driving innovation and efficiency in numerous fields.
Transfer Learning from Large Language Models
The intersection of transfer learning and large language models, like GPT-3, marks a significant milestone in AI. These models, trained on extensive datasets, encompass a broad spectrum of human language and knowledge. In the context of an AI development company, the potential to fine-tune these models for specific applications opens up a myriad of possibilities.
One key application is in creating more sophisticated chatbots and virtual assistants. By fine-tuning large language models, companies can develop AI systems that understand and respond to user queries with unprecedented accuracy and context-awareness. This is especially important for businesses looking to enhance customer engagement and automate customer service processes.
Another area where these models shine is in content generation. Whether it’s drafting articles, creating marketing copy, or even composing music, the ability of these models to generate coherent and contextually relevant content is a game-changer. This aspect is particularly aligned with generative AI services, where the aim is to produce original and creative outputs based on existing data patterns.
The use of large language models in transfer learning is not just about their current capabilities, but also about their potential to evolve and adapt as they are exposed to more specific and varied datasets. This adaptability is what makes them a cornerstone in the field of AI and machine learning, offering robust solutions across diverse sectors.
Transfer Learning Services
In the realm of transfer learning services, the focus is on leveraging pre-existing AI models and adapting them to new, specific tasks or datasets. This service is crucial for businesses looking to implement AI without the need for extensive data or resources required to train models from scratch.
Transfer learning services can encompass various aspects, from initial consultation and model selection to data preparation, model training, and ongoing support. For an AI development company seeking AI integration services, these offerings provide a streamlined pathway to integrate AI into their existing systems and processes.
For instance, a company with a substantial database of customer interactions may want to improve their recommendation systems. Transfer learning services can adapt a pre-existing model to this dataset, enhancing the system’s accuracy and relevance to their customer base.
The benefit of these services is clear: they provide a cost-effective, efficient, and accessible way for businesses to harness the power of AI. By reducing the barriers to entry, transfer learning services democratize access to advanced AI technologies, enabling businesses of all sizes to benefit from the transformative potential of AI.
Transfer of Learning
The concept of ‘transfer of learning’ extends beyond AI and machine learning into broader educational and organizational contexts. It’s about applying knowledge, skills, and competencies acquired in one context to new contexts. This principle is integral in education systems, workforce training, and professional development, where the ability to adapt and apply learned skills in various situations is crucial.
In educational settings, transfer of learning is key to how students apply theoretical knowledge to practical situations. For instance, learning the principles of physics in a classroom and then applying them in real-world engineering problems. This approach is vital for developing critical thinking and problem-solving skills, essential in today’s ever-changing job market.
In the corporate world, transfer of learning is central to employee training and development programs. Businesses invest in training programs for specific job skills and to enhance employees’ ability to adapt them to different roles or challenges. This is where technology consulting services play a significant role. They offer expertise in developing training programs that effectively facilitate the transfer of learning, ensuring that employees can adapt and apply their skills in various technological and business contexts.
The efficacy of transfer of learning is measured by how well skills and knowledge are applied in new situations, which is a crucial indicator of the quality of training and education. As the workplace and educational environments continue to evolve, the importance of transfer of learning grows, emphasizing the need for adaptable, lifelong learning skills.
Integration with Generative AI Services
Generative AI services, which encompass the creation of new, unique content through AI models, have been significantly enhanced by transfer learning. In these services, models pre-trained on a vast array of data – from text to images – are fine-tuned to generate specific types of content. This is particularly impactful in fields like advertising, where creating original and engaging content is key.
For instance, in graphic design, transfer learning enables generative AI services to produce unique visual content that aligns with a brand’s aesthetics and messaging. Similarly, in content writing, these services can generate articles or marketing copy that resonates with a specific audience, all while maintaining a consistent tone and style.
The application of transfer learning in generative AI services demonstrates its ability to not only create new content but also ensure that this content is tailored and relevant. This capability is essential for businesses looking to stand out in a crowded digital landscape with original and compelling AI-generated content.
Role in Digital Transformation Services
Transfer learning plays a crucial role in digital transformation services, acting as a catalyst in the integration and optimization of AI within businesses. In digital transformation initiatives, transfer learning accelerates the adoption of AI by enabling businesses to leverage existing models and quickly adapt them to their specific needs and challenges.
This approach is particularly beneficial for organizations that are transitioning from traditional operational models to more data-driven and AI-integrated processes. By utilizing transfer learning, these businesses can rapidly implement AI solutions, such as predictive analytics or automated customer service systems, without the need for extensive data collection and model training from scratch.
In essence, transfer learning is a key enabler in digital transformation, helping businesses to navigate the complexities of AI implementation and harness its benefits more efficiently and effectively. This accelerates the transformation process, allowing companies to rapidly adapt to the evolving digital landscape and stay competitive.
Contribution to Technology Consulting Services
Transfer learning significantly contributes to technology consulting services, particularly in guiding businesses through AI and machine learning implementation. It plays a crucial role in developing AI strategies that are both efficient and effective, tailored to the unique needs of each business.
Technology consultants utilize transfer learning to advise companies on how to leverage pre-trained models, thereby avoiding the high costs and time associated with training models from scratch. This is particularly beneficial for small to medium-sized enterprises (SMEs) or startups that may have limited data or resources for AI development.
Consultants also use transfer learning to help businesses integrate AI into existing processes seamlessly. This involves identifying areas within a business where AI can be most beneficial and then adapting AI models to fit these specific applications. For instance, consultants might assist a retail company in implementing AI for personalized customer recommendations or help a manufacturing company use AI for predictive maintenance.
Moreover, transfer learning is key in ensuring that AI solutions remain adaptable and scalable. As a business grows and evolves, its AI models need to adapt as well. Transfer learning provides the flexibility to retrain these models on new data or for different purposes, a critical aspect covered in technology consulting services.
By leveraging transfer learning, technology consultants provide businesses with the tools and knowledge to harness the full potential of AI, ensuring they stay competitive in an increasingly digital world.
Custom Machine Learning Solutions Powered by Transfer Learning
Custom machine learning solutions, particularly those enhanced by transfer learning, offer businesses tailored approaches to meet their specific challenges and goals. These solutions leverage the power of pre-trained AI models, adapting them to address unique business problems or to capitalize on specific opportunities.
For instance, in the healthcare sector, custom solutions can involve adapting models trained on general medical data to focus on rare diseases, enabling more accurate diagnoses and treatment plans. In the retail sector, these solutions can personalize customer experiences by fine-tuning recommendation systems based on individual purchasing behaviors and preferences.
The advantage of custom machine learning solutions lies in their ability to provide highly targeted and effective AI implementations. By utilizing transfer learning, the development time and costs are significantly reduced, as the core AI model has already been trained on extensive datasets. Businesses can then focus on fine-tuning these models with their data, ensuring that the final AI solution is closely aligned with their specific requirements.
Moreover, these custom solutions offer scalability and adaptability. As a business grows and its data evolves, the AI models can be retrained or further adapted to meet these changing needs. This ensures that the AI solutions remain relevant and continue to provide value over time.
In summary, custom machine learning solutions empowered by transfer learning represent a strategic advantage for businesses in various industries. They offer a pathway to harness advanced AI capabilities in a cost-effective, efficient, and highly targeted manner, driving innovation and competitive advantage in the marketplace.
Conclusion: Embracing the Potential of Transfer Learning
As we reach the conclusion of our exploration into transfer learning and its myriad applications, it’s clear that this technology is not just a component of modern AI; it’s a driving force behind numerous innovations across various industries. From enhancing healthcare outcomes through personalized medical analysis to revolutionizing retail experiences with tailored customer engagement, transfer learning stands at the forefront of AI development.
For businesses seeking to harness the power of AI, the journey towards integrating transfer learning into their operations can be transformative. By leveraging the knowledge and capabilities of pre-trained models, companies can achieve significant advancements in efficiency, accuracy, and innovation, all while reducing development time and costs.
In a world where digital transformation is key to business success, transfer learning offers a pathway to not just compete but to lead in an increasingly data-driven landscape. As an AI development company specializing in custom machine learning solutions, the potential to tailor these advanced AI capabilities to specific business needs and challenges is immense.
Transfer learning is more than just a technological trend; it’s an essential strategy for any organization looking to capitalize on the opportunities presented by AI and machine learning. As we move forward into an increasingly AI-integrated future, the role of transfer learning in driving business growth, innovation, and efficiency will only continue to expand.
This comprehensive exploration of transfer learning showcases its pivotal role in various sectors and highlights how businesses can leverage this technology to achieve their specific objectives. For more insights and assistance in implementing these AI-driven solutions, consider partnering with an AI development company specializing in custom machine learning solutions. Embrace the power of AI and propel your business towards new heights of innovation and success.
FAQs: Transfer Learning in AI and Machine Learning
Q1. What is Transfer Learning and How Does it Benefit AI Development?
Transfer learning involves applying knowledge gained from one problem to a different but related problem. It benefits AI development by reducing the need for extensive data collection and training time, making AI model development faster and more efficient.
Q2. Can Transfer Learning Be Applied Across Different Industries?
Yes, transfer learning is versatile and can be applied across various industries like healthcare for predictive diagnostics, retail for personalized customer experiences, finance for fraud detection, and many others, enhancing the effectiveness of AI solutions in these sectors.
Q3. How Does Transfer Learning Reduce AI Development Costs?
Transfer learning reduces AI development costs by utilizing pre-trained models that require less data and computational resources to fine-tune for specific tasks, thereby cutting down on the time and expense associated with developing models from scratch.
Q4. What Role Do AI Development Companies Play in Transfer Learning?
AI development companies specialize in identifying and customizing pre-trained models for specific business needs. They provide expertise in adapting these models to new datasets or tasks, ensuring effective and tailored AI solutions for their clients.
Q5. Are There Any Challenges Associated with Implementing Transfer Learning?
Challenges in transfer learning include ensuring data compatibility between the pre-trained model and the new task, managing the balance between model adaptability and overfitting, and requiring domain-specific knowledge to effectively fine-tune models for specific tasks.