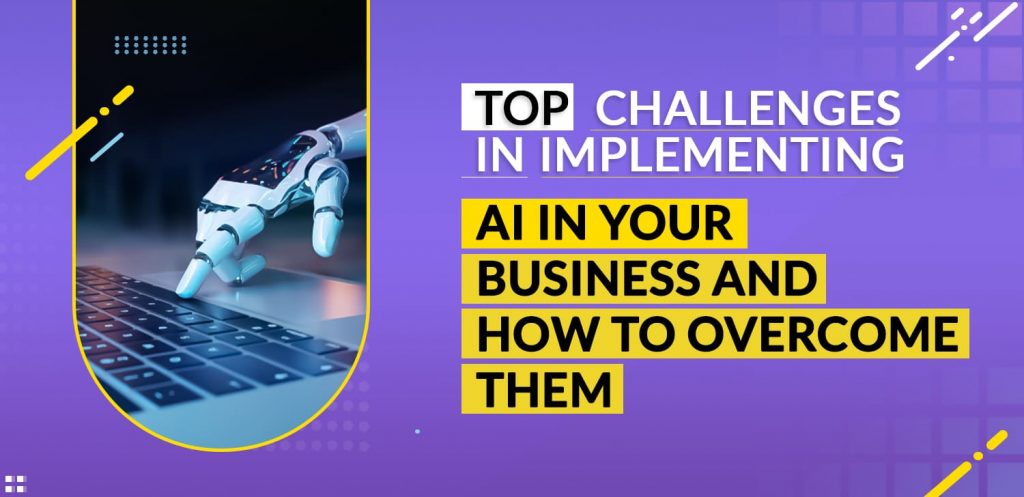
Process optimization, fraud detection, research capabilities, and a lot more: AI brings a wide array of benefits for businesses, regardless of their industry and size. With AI’s maturity, the systems are only becoming more efficient, capable and transforming the global community for the better.
Various tech giants and industry leaders have identified AI as a game-changer and believe that now is the time to invest in technology to help your business. However, multiple challenges and debates concerning AI implementation still force brands to stand at the fence. Let’s discuss some of these issues that keep AI experts and businesses up at night while talking about the ways to resolve them.
1. Technology Roadmap
AI has been around for decades now. However, popular AI solutions such as voice assistants, face swap applications, self-driving cars, and more became common only a couple of years ago. Businesses and AI engineer brands do not yet have the right set of guidelines and roadmap to implement AI solutions that lead to the following technological challenges.
a. Poor Architecture Choice
Challenge
When it comes to AI, there is no one size fits all approach. AI is not just there to forecast future trends. There are multi-tenant applications that serve a global userbase of over 1000s of users. These applications require high performance, scalability, and better quality of management. AI developers cannot simply write a Flask service, put it in a docker container and deploy the AI model. While this may work great for some systems, it may hamper the performance for specific applications when it hits the limit. The only result is another non-working application that fails to deliver.
Solution
The right way to build AI architecture is to collaborate with an expert AI development company and create the proper roadmap. The steps required to be followed in order to choose the accurate architecture include:
- Define AI strategy
- Prepare AI data with expert data scientists
- Find the right technology alternative for AI data management and governance
- Build the data architecture
- Train and develop machine learning models
Apart from this, businesses and technology partners should also consider the following factors:
- Flexible Storage: Design an efficient, organization-wide storage solution that satisfies the data requirements and is scalable to meet changing business demands. While checking for options, shortlist according to the data structure, digital footprint, and usage.
- Powerful Computation: A robust, scalable, and secure computing infrastructure allows data experts to implement data preparation techniques. It also enables models to work flawlessly to find the solution with a quick turnaround.
- Elasticity: Storage and resource consumption for AI models can be dynamic. Elastic infrastructure enables optimal usage of limited computation resources.
b. Wrong or Insufficient Training Data
Challenge
AI-based systems are as accurate as the data fed into them. However, it is not always possible for the companies and technology partners to have the correct data set in the real-world scenario. Every firm has confidential pieces of information that are not accessible to everyone. For instance, Healthcare data is not available for everyone due to privacy reasons. In such cases, only a small amount of data is available to train the ML model, which leads to inaccurate outcomes. Two types of issues that can arise include:
- Over-fitting: The training model would read too much of the data for too little. This implies training model memorizes the patterns, which also leads to low training errors and high-test errors.
- Under-fitting: It would build an extremely simple model that is not trained to deliver the right results. Model is unable to capture the relationship in data with zero predictive power.
Solution
Collecting data by yourself is not a great solution to this issue. Data scientists across the globe handle this challenge using one or more of the following methods.
- Data simulation
- Manual data labeling
- Synthetic data generation
2. Lack of AI Explainability
Challenge
Explainable Artificial Intelligence (XAI) is a set of processes that enable humans to comprehend and trust the results and output delivered by machine learning algorithms. It describes an AI model and its expected outcomes as well as potential biases. Helping deduce the accuracy, fairness, transparency, and expected results of the AI-based systems, XAI is vital for organizations to build trust and confidence during deployment.
While uncovering potential issues, XAI enables organizations to adopt a careful approach to AI implementation initiatives.
AI explainability also helps an organization adopt a responsible approach to AI development. Unlike black-box models like neural networks, XAI enables a model that allows you to map the solution requirements against business needs, address technology challenges, and build a system architecture that delivers the right scalability. However, owing to various reasons, businesses fail to implement AI explainability in its true sense. Here is how to solve it.
Solution
Next-gen AI structures are expected to have hierarchical meta-learning capabilities that can adapt to the diverse environment by establishing a cause-effect relationship. Efforts need to focus on creating flexible and interpretive models that can deduce results based on multiple aspects. It is also vital to ensure data relevance and its accurate evaluation.
3. Scaling Artificial Intelligence
Challenge
Scalable AI defines the AI solutions that are accurate as well as robust. Here, robust is used to describe the ability of AI solutions to seamlessly meet the requirements of various companies or users, contexts and situations, and geographies.
AI scalability is not challenging as a part of technology itself, but how AI is implemented for commercial applications. It requires a fundamental paradigm shift in the development process.
Owing to this, commercial AI products fail to scale as intended, leading to fatigue for the and the business stakeholders. AI fatigue is caused by the high level of hype and high volume of information, sometimes inaccurate information about what the system can achieve. For most of such applications, AI products fail to deliver during commercial use.
Solution
Studies show that the brands that can industrialize AI at scale invest in people and processes as much as they do in technology. Most of them use the following three mantras to scale AI:
- They set the direction and follow the course: AI is a step-by-step process that requires vision, strategy, and roadmap. It would be best if you had sustained commitment to these to achieve the results.
- They have laid out the guidelines for AI development and implementation: AI-powered applications have a typical lifecycle from POC to tested prototype or MVP and then the full-fledged product. A proven approach to doing this can help build scalable AI solutions.
- They have the right technology stack: Companies that use standardized platforms can leverage rapid development robustly and sustainably.
4. Replicating Lab Results in Real Life
Challenge
Nature published a response written by 31 scientists to a study by Google health that appeared in the journal last year. Google described its successful trials of an AI solution that looked for signs of breast cancer in medical images. However, critics suggested that Google provided less information about the code and tested that it amounted to nothing but a mere promotion of the proprietary technology.
Similarly, Oxford and Google DeepMind scientists developed a deep neural network that read people’s lips with 93% accuracy. In the current scenario, AI-based systems can also detect COVID-19 in asymptomatic patients based on cough recorded on cellphones. However, this replication of the lab results in proper form is only possible with the combination of powerful hardware and correct training data.
However, the lack of transparency prevents new AI models and techniques from being assessed on the metrics like robustness, bias, and security. AI solutions quickly transition to real-world from research labs. As it directly impacts people’s lives, it can be challenging as solutions that work seamlessly in labs fail in real life.
Solution
The solution to this problem is extensive testing. Every innovation in the field of AI requires industry leaders to build upon and improve the same. This helps build the correct data set and implements a model that evolves with time, thereby delivering expected results.
5. AI Ethical Issues
Challenge
With the popularity of AI, products come multiple AI ethical issues. These mainly include:
- Biased algorithmic decision-making that arises from flawed training data prepared manually and can contain social and historical inequities.
- Moral implications mainly revolve around companies’ intent to replace humans with productive robots.
The best example of these can be seen in AI solutions that inherit racial and gender prejudice from their developers. A facial recognition system deployed by US law enforcement agencies is likely to identify a non-white person as a criminal. However, these problems are easier to solve.
Solution
Companies need to create balanced training datasets that include facial images of diverse races, gender, age, and sexual orientation. This can lead to some of the most efficient AI products like AI-powered HR management software that can scan the CV of the applicants and identify potential candidates based on specific parameters.
The Bottom-line
AI has been evolving, and so are the challenges that come with the implementation. However, with innovation paving its way into the mainstream, organizations need to understand these issues at the core and address them during the development and ideation phase. The solutions can be summarized as:
- Strengthen consumer data as well as protection measures
- Establish a general shared framework and set of principles for AI usage
- Ensure best practices to address the issues such as security, bias, and explainability
- Strike the right balance between business and competitive edge to build AI solutions to ensure AI benefits are widely available.
With all this, you also need a reliable AI development partner by your side who does not just have the right expertise but also holds industry experience that enables them to understand these challenges at the core. At Matellio, we offer expert AI development services guided by best principles, work ethics, and years of experience. Our development process takes care of everything right from ideation to prototype development to MVC and product deployment. Book a free consultation with our team right away to begin now.