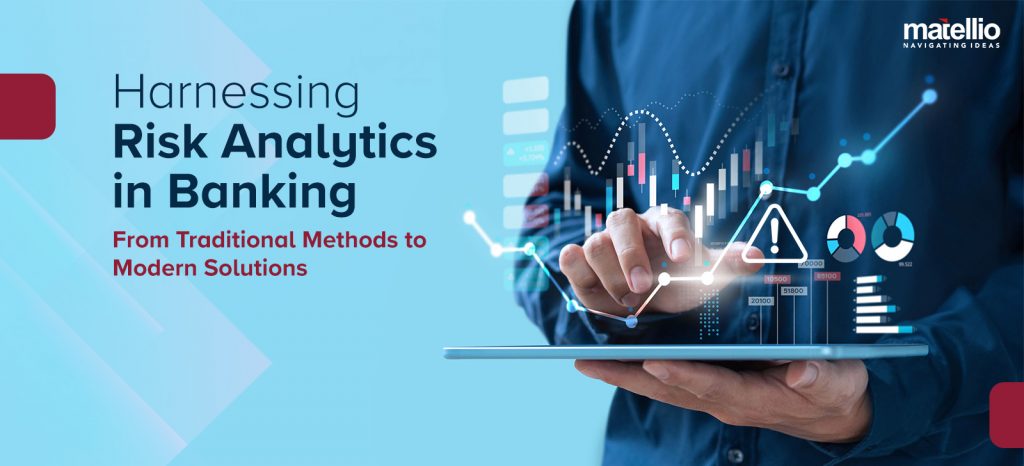
Risk management is a cornerstone of the banking industry, ensuring financial stability and safeguarding against potential losses. Effective risk management protects banks from various uncertainties, from credit defaults to market fluctuations, maintaining trust among stakeholders and customers.
Risk analytics in banking, a modern approach to risk management, leverages data-driven techniques to assess, predict, and mitigate risks more accurately and efficiently. Its growing significance in banking lies in its ability to provide deep insights, enabling banks to make informed decisions, comply with regulations, and enhance overall operational efficiency.
What Is Risk Analytic in Banking?
Risk analytics in banking refers to the application of advanced analytical techniques and technologies to identify, assess, and manage various types of risks faced by financial institutions. It involves the use of data analysis, statistical models, and machine learning algorithms to evaluate potential risks, forecast their impact, and support decision-making processes to mitigate those risks effectively.
Evolution from Traditional Risk Management to Modern Analytics in Banking
The evolution from traditional risk management to modern analytics involves significant technological advancements that have transformed how organizations identify, assess, and mitigate risks. This evolution can be traced through several key technical aspects:
Data Collection and Integration
Traditional Approach:
- Relied heavily on manual data collection methods, including surveys, audits, and historical records.
- Data silos were common, with different departments maintaining separate databases, leading to fragmented risk information.
Modern Analytics:
- Utilizes automated data collection through IoT devices, sensors, and APIs.
- Integration of various data sources into centralized data lakes and warehouses using ETL (Extract, Transform, Load) processes.
Data Processing and Storage
Traditional Approach:
- Data processing was largely manual or semi-automated, often using spreadsheets and basic database systems.
- Limited storage capacity constrained the amount of data that could be retained and analyzed.
Modern Analytics:
- Advanced data processing frameworks, including distributed computing and parallel processing, enable real-time data analysis.
- Cloud-based storage solutions (e.g., AWS S3, Google Cloud Storage) provide scalable and cost-effective storage options.
- Implementation of data lakes and data warehouses (e.g., Amazon Redshift, Snowflake) for efficient data management and querying.
Risk Identification and Assessment
Traditional Approach:
- Risk identification was primarily qualitative, based on expert judgment and predefined checklists.
- Risk assessments were periodic and often lagged actual risk events.
Modern Analytics:
- Machine learning (ML) and artificial intelligence (AI) algorithms identify patterns and anomalies in real time.
- Predictive analytics models (e.g., regression analysis, neural networks) forecast potential risks based on historical data.
- Natural language processing (NLP) techniques analyze textual data from news feeds, social media, and other sources to identify emerging risks.
Risk Monitoring and Reporting
Traditional Approach:
- Risk monitoring was manual, with periodic reviews and static reports.
- Reporting relied on static charts and graphs, often outdated by the time they were reviewed.
Modern Analytics:
- Real-time dashboards and visualization tools (e.g., Tableau, Power BI) provide dynamic and interactive risk monitoring.
- Automated alerts and notifications inform stakeholders of risk events as they occur.
- Advanced analytics platforms integrate with business intelligence tools for comprehensive and up-to-date reporting.
Also Read: Streamlining Compliance and Risk Management in Banking through RPA
Decision Support and Mitigation
Traditional Approach:
- Decision-making was largely subjective, based on experience and intuition.
- Mitigation strategies were reactive, addressing risks after they had materialized.
Modern Analytics:
- Decision support systems (DSS) leverage advanced analytics to provide data-driven insights and recommendations.
- Prescriptive analytics models (e.g., optimization algorithms and scenario analysis) suggest proactive mitigation strategies.
- Integration with enterprise risk management (ERM) systems for coordinated and strategic risk response.
Cybersecurity Risk Management
Traditional Approach:
- Cybersecurity risk management was basic, focusing on perimeter security and reactive threat response.
- Limited use of advanced tools for threat detection and incident response.
Modern Analytics:
- Advanced threat detection leveraging AI integration services and ML models that analyze network traffic and user behavior.
- Encryption, multi-factor authentication (MFA), and other advanced security measures are used to protect data integrity and confidentiality.
Key Benefits of Implementing Risk Analytics in Banking
Data-Driven Insights
Risk analytics in banking employs advanced data processing techniques such as data mining, NLP, and big data analytics to analyze extensive volumes of both structured (e.g., transaction records, financial statements) and unstructured data (e.g., social media, news articles). This method enhances the ability to identify trends, patterns, and correlations that might be overlooked through manual methods.
Predictive Modeling
Through machine learning algorithms like regression analysis, decision trees, and neural networks, risk analytics in financial services helps forecast potential risks and outcomes. By utilizing historical data and real-time information, banks can predict future events and take proactive measures to mitigate risks.
Enhanced Financial Performance
The implementation of risk analytics in banking leads to improved decision-making, which enhances overall financial results by optimizing investment portfolios, reducing losses, and increasing returns. It aids in balancing risk and reward, ensuring that capital allocation is optimized based on risk-adjusted returns.
Continuous Monitoring
Risk analytics tools enable continuous monitoring systems that track compliance with regulatory requirements in real time. By utilizing compliance management software, banks can automate the monitoring process, ensuring adherence to all regulatory requirements without manual intervention. This integration of digital transformation services enhances constant oversight, reducing the risk of non-compliance by providing immediate detection of deviations from regulatory standards.
Automated Alerts
Setting up automated alerts and notifications through risk analytics systems informs compliance officers of potential non-compliance issues as they arise. Integrating these alerts with existing IT systems ensures that notifications are timely and relevant, allowing for swift corrective actions. This reduces reliance on manual checks, increasing the speed and accuracy of compliance monitoring and ensuring a proactive approach to managing regulatory risks.
Regulatory Change Management
Analytics play a vital role in interpreting regulatory changes and assessing their impact on the organization’s operations and risk profile. Using rule-based engines and AI, banks can automatically update compliance protocols in response to new regulations. Developing adaptive compliance strategies that evolve with regulatory updates ensures ongoing adherence and reduces the risk of non-compliance, which is vital for safeguarding the institution’s reputation.
Reduced Penalties
Proactively identifying and addressing compliance gaps through risk management analytics helps minimize the risk of incurring fines and penalties. This proactive approach enhances the organization’s reputation with regulators and stakeholders by demonstrating a commitment to compliance, thus reducing the financial and reputational damage associated with regulatory breaches.
Early Threat Detection
By utilizing real-time data analytics and anomaly detection algorithms, banks can monitor both internal and external data sources for early warning signs of risk, such as unusual transaction patterns or cybersecurity threats. This proactive approach enhances the organization’s ability to respond quickly to emerging risks and minimize their impact.
Predictive Analytics
Using predictive models to forecast and mitigate risks supports preemptive action and better risk management. Developing these models based on historical data and current trends allows banks to anticipate future risk events and supports the creation of proactive risk mitigation strategies. This predictive capability is crucial for managing uncertainties and enhancing risk management practices in the banking sector.
Enhanced Security
By addressing identified vulnerabilities and implementing robust risk mitigation measures, risk analytics strengthens the overall security posture. Advanced security analytics help detect and respond to potential threats in real time, protecting sensitive data and financial assets from potential threats through proactive risk management. This approach is essential for maintaining a secure banking environment.
Minimized Losses
It aids in reducing financial losses by preventing risky events before they occur through early identification and mitigation. Implementing risk controls and contingency plans helps mitigate the impact of identified risks, enhancing the organization’s resilience and minimizing disruption and loss. Effective risk management practices are fundamental for reducing potential financial impacts in banking.
Process Automation
By streamlining processes through robotic process automation (RPA) and other automation technologies, risk analytics in banking reduce manual interventions. Automating repetitive and time-consuming tasks increases the speed and accuracy of risk management processes, freeing up staff to focus on higher-value activities and reducing the likelihood of errors. This automation is key to achieving operational efficiency in banking.
Cost Savings
The implementation of risk analytics in banking leads to significant operational cost reductions by minimizing the need for manual labor and decreasing errors associated with manual processes. Greater efficiency in risk management operations lowers costs and reduces the financial burden of compliance through automated monitoring and reporting processes.
Resource Optimization
Risk analytics helps optimize resource allocation by identifying areas where automation and efficiency improvements can be made. Ensuring that human resources focus on high-value tasks enhances overall productivity by streamlining workflows and reducing bottlenecks.
Tailored Products and Services
It enables the customization of financial products and services to meet the specific demand and preferences of individual customers. By offering personalized solutions, banks enhance customer satisfaction and build targeted marketing campaigns based on customer insights.
Creditworthiness Assessment
Accurately evaluating customer creditworthiness through risk analytics in banking involves analyzing comprehensive data such as credit scores, transaction history, and financial behavior. This approach reduces the risk of loan defaults and enhances the ability to offer credit products to a wider range of customers, including those with non-traditional credit histories.
Types of Risk Analytics in Banking
Credit Risk Analytics
Credit risk analytics involves assessing the likelihood that borrowers will default on their obligations, resulting in financial loss to the bank.
Components
- Credit Scoring Models: Use statistical techniques to evaluate a borrower’s creditworthiness by analyzing factors such as credit history, income levels, and debt-to-income ratios.
- Loss Given Default (LGD): Predicts the amount of loss the bank would incur if the borrower defaults, considering factors like collateral and recovery rates.
- Exposure at Default (EAD): Measures the total value exposed to default at the time of the borrower’s default.
Applications
- Loan Approval and Pricing: Helps in determining whether to approve a loan and what interest rate to charge based on the assessed risk.
- Portfolio Management: Enables banks to manage their loan portfolios by identifying high-risk loans and making necessary adjustments.
- Regulatory Compliance: Ensures adherence to regulatory requirements such as Basel III, which mandates banks to maintain adequate capital against potential credit losses.
Market Risk Analytics
It focuses on evaluating the risk of losses from fluctuations in market prices for both on- and off-balance sheet positions.
Components
- Value at Risk (VaR): Estimates the maximum potential loss over a specified period with a given confidence interval.
- Stress Testing: Simulates extreme market conditions to evaluate the bank’s resilience to adverse market movements.
- Scenario Analysis: Examines the impact of hypothetical scenarios on the bank’s financial position.
- Sensitivity Analysis: Measures how changes in market variables (e.g., interest rates, foreign exchange rates) affect the value of assets and liabilities.
Applications
- Trading and Investment Decisions: Guides traders and portfolio managers in making informed decisions to mitigate market risks.
- Risk Mitigation Strategies: Helps in developing hedging strategies to protect against potential market fluctuations.
- Regulatory Reporting: Facilitates compliance with regulatory requirements such as those stipulated by the Dodd-Frank Act and the European Market Infrastructure Regulation (EMIR).
Operational Risk Analytics
It involves assessing the risk of loss resulting from inadequate or failed internal processes, people, systems, or external events.
Components
- Risk Control Self-Assessment (RCSA): A systematic process for identifying and assessing risks and the effectiveness of controls in place.
- Key Risk Indicators (KRIs): Metrics used to provide early signals of increasing risk exposures in various areas of operations.
- Loss Event Database: A collection of data on past operational risk events to help in analyzing and forecasting future risks.
- Scenario Analysis: Evaluate the impact of potential future operational risk events.
Applications
- Fraud Detection and Prevention: Utilizes analytics to identify unusual patterns that may indicate fraudulent activities.
- Process Improvement: Identifies weaknesses in operational processes and suggests improvements to reduce risk.
- Regulatory Compliance: Ensures compliance with regulations such as the Sarbanes-Oxley Act (SOX) and the Basel II and III frameworks.
Liquidity Risk Analytics
Liquidity risk analytics assess the risk that a bank will not be able to meet its short-term financial obligations due to an inability to convert assets into cash without significant losses.
Components
- Liquidity Gap Analysis: Evaluate the difference between a bank’s liquid assets and its short-term liabilities over different time horizons.
- Liquidity Coverage Ratio (LCR): Measures the bank’s ability to withstand a 30-day stressed funding scenario by comparing high-quality liquid assets to total net cash outflows.
- Cash Flow Forecasting: Projects future cash inflows and outflows to ensure sufficient liquidity.
Applications
- Asset-Liability Management (ALM): Helps in managing the bank’s balance sheet to ensure a proper match between assets and liabilities.
- Contingency Funding Plans: Develop strategies for securing alternative funding sources in times of liquidity stress.
- Regulatory Compliance: Assists in meeting regulatory liquidity requirements set by Basel III and other frameworks.
Cyber Risk Analytics
Cyber risk analytics assesses the potential for losses due to cyber threats and attacks, including data breaches, hacking, and other cyber incidents.
Components
- Threat Intelligence: Collects and analyzes data on emerging cyber threats and vulnerabilities.
- Vulnerability Assessment: Identifies and evaluates weaknesses in the bank’s IT infrastructure that could be exploited by cyber attackers.
- Incident Response Analytics: Analyzes data from past cyber incidents to improve response strategies and reduce recovery times.
- Cyber Risk Scoring: Assigns risk scores to various IT assets and processes based on their susceptibility to cyber threats.
Applications
- Risk Mitigation Strategies: Helps in developing comprehensive cyber risk management plans that include preventive, detective, and corrective controls.
- Compliance: Ensures adherence to cybersecurity regulations such as the General Data Protection Regulation (GDPR) and the Cybersecurity Information Sharing Act (CISA).
- Training and Awareness: Uses analytics to identify areas where employee training and awareness programs can be improved to reduce cyber risks.
Wish To Harness the Power of Risk Analytics for Any of These Applications?
Connect With Us!
Advanced Technologies Driving Risk Analytics in Banking
Machine Learning and AI
- Machine learning and AI in banking algorithms can analyze vast amounts of structured and unstructured data to identify complex patterns and relationships that traditional statistical methods might miss.
- These technologies enhance the accuracy of predictive models, enabling banks to foresee potential risks and take preemptive actions.
- AI can continuously learn from new data, refining its predictions over time and adapting to changing risk environments.
- AI and machine learning can detect unusual transaction patterns indicative of fraudulent activities, flagging them for further investigation.
- These systems can adapt to new fraud tactics, offering robust protection against evolving threats.
Big Data Analytics
- Big data analytics leverages extensive datasets from various sources, including transactional data, market data, social media, and customer feedback, to provide a comprehensive view of risk factors.
- By incorporating diverse data sources, big data analytics enhances the accuracy of risk models, leading to more reliable risk assessments.
- Big data technologies enable real-time risk analysis, allowing banks to respond quickly to emerging threats and opportunities.
Also Read: Big Data Analytics: Unlocking Industry-Specific Solutions for Transformative Business Insights
Cloud Computing
- Cloud computing provides scalable analytics solutions, allowing banks to efficiently handle and analyze large volumes of data without substantial upfront investments in physical infrastructure.
- By leveraging cloud integration services, banks can dynamically allocate resources based on current needs and workloads. This flexibility ensures that the infrastructure adapts seamlessly to changes in demand and operational requirements.
- Cloud platforms facilitate improved collaboration among different departments and locations. By integrating data and insights through cloud-based systems, banks can enhance coordination in their risk management efforts.
Robotic Process Automation (RPA)
- RPA automates repetitive and time-consuming tasks in risk management processes, such as data entry, report generation, and compliance checks.
- By automating routine tasks, RPA reduces labor costs and frees up human resources for more strategic activities.
- RPA ensures consistent execution of compliance tasks, reducing the risk of non-compliance due to human oversight.
- RPA can integrate data from various sources, ensuring that risk management analytics have access to complete and up-to-date information.
Also Read: The Future of Banking – How RPA is Shaping Digital Transformation in Banks
Internet of Things (IoT)
- IoT devices gather real-time data from various sources, such as ATMs, branch networks, and customer devices, providing dynamic and up-to-date risk assessments.
- This real-time monitoring allows for immediate detection and response to emerging risks.
- IoT analytics can predict maintenance needs for critical banking infrastructure, reducing downtime and preventing potential operational risks.
- IoT devices enhance security by monitoring physical and digital environments, detecting unusual activities, and triggering alerts.
Get a Free Consultation on Implementing Risk Analytics in Your Bank
Fill out The Form!
Challenges and Solutions in Implementing Risk Analytics in Banking
Data Quality and Integration
Challenge: Ensuring accurate and comprehensive data across multiple sources. Banks often deal with data from various internal and external sources, leading to inconsistencies and inaccuracies. Integrating data from disparate systems can be complex and time-consuming, resulting in potential data quality issues.
Solution: Implementing robust data governance frameworks and utilizing data integration tools to maintain high data quality and consistency is essential. Establishing clear data governance policies helps standardize data formats and ensure accuracy. Additionally, leveraging advanced data integration tools that automate the consolidation of data from multiple sources improves consistency and reliability. Engaging in data consultation services can further enhance this process by providing expert guidance on best practices for data management and integration, ensuring that the frameworks and tools are effectively aligned with the bank’s specific needs and goals.
Regulatory Compliance
Challenge: Navigating complex and evolving regulatory landscapes. Regulations in the banking sector are constantly changing, making it challenging to stay compliant. Non-compliance can lead to significant penalties and damage to reputation.
Solution: Leveraging advanced compliance monitoring tools and staying updated with regulatory changes to ensure continuous adherence. Implement real-time compliance monitoring systems that automatically track and report compliance status. Stay informed about regulatory updates and integrate regulatory change management into the risk management analytics process.
Cost of Implementation
Challenge: Balancing investment with long-term benefits. Implementing risk analytics can require significant initial investment in technology and infrastructure. Banks need to justify the costs with tangible long-term benefits.
Solution: Opt for scalable, cloud-based solutions that offer flexibility and lower upfront costs, ensuring a gradual and cost-effective implementation. Choose cloud-based analytics solutions that provide scalability and reduce the need for large upfront capital expenditure. Implement risk analytics in phases, allowing for gradual investment and easier demonstration of benefits over time.
Data Privacy and Security
Challenge: Protecting sensitive customer data from breaches and ensuring compliance with data privacy regulations. The banking sector handles vast amounts of sensitive customer information, making it a prime target for cyberattacks.
Solution: Implementing robust cybersecurity measures, encryption, and regular audits to safeguard data integrity and privacy. Use advanced encryption methods to protect data both in transit and at rest. Conduct regular security audits and vulnerability assessments to identify and address potential threats.
Beat These Challenges and Secure Your Business with Custom-Built Risk Analytics Solutions!
Contact Us
How Can Matellio Help with Risk Analytics in Banking?
Matellio offers comprehensive solutions for risk analytics in banking, tailored to unique needs. Our approach integrates cutting-edge technologies and industry expertise to address the unique challenges faced by banks.
- We begin by assessing your existing systems and requirements, utilizing our extensive technology consulting services to tailor a risk analytics solution that aligns with your specific needs.
- Our team specializes in developing and implementing risk models that leverage data-driven insights to identify and mitigate potential risks effectively.
- Our services include continuous support and maintenance to ensure that your risk analytics system operates smoothly and adapts to evolving regulatory standards.
- We also provide secure data migration strategies to transition seamlessly to new risk analytics solutions, protecting data integrity and minimizing disruption.
- With our expertise in risk analytics, your bank can achieve better decision-making, enhanced compliance, and improved risk management, ultimately strengthening your overall financial stability and operational efficiency.
You can fill out the form and reach out for our expert’s guidance to explore how you can upgrade your risk management operations.
FAQ’s
Q1. Can risk analytics in banking integrate with existing systems?
Yes, risk analytics can integrate with both legacy and modern systems. We create hybrid solutions to enhance your current IT infrastructure, ensuring a smooth transition and continuity.
Q2. What are the costs associated with implementing risk analytics in banking services?
Costs vary based on risk model complexity, technology needs, and integration. We provide transparent estimates tailored to your budget and objectives.
Q3. What support and maintenance do you offer for risk analytics in banking?
We offer 24/7 support, regular updates, performance optimization, and proactive monitoring to keep your system efficient and up to date.
Q4. How is data migration managed during the implementation of risk analytics in banking?
We use a secure, structured approach to data migration with thorough planning and testing. Backup and recovery solutions ensure data integrity and minimal disruption.
Q5. Can risk analytics in banking solutions be customized to meet specific organizational needs?
Yes, our solutions are customizable to fit your unique risk management needs, including specific models and workflows.
Q6. How does Matellio ensure data security and compliance in risk analytics solutions?
We implement encryption, access controls, and regular audits to ensure data security and regulatory compliance, protecting sensitive information and maintaining risk management integrity.