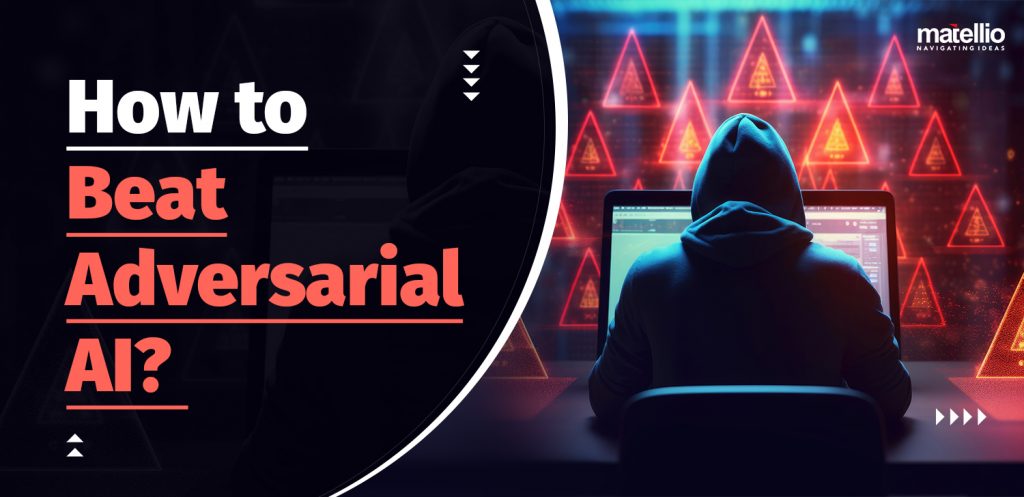
In an era where digitalization and technology are rapidly permeating every sector, their transformative power is undeniable. From streamlining operations to revolutionizing industries, these advancements offer unprecedented efficiency and innovation. However, akin to a coin with dual faces, these technologies cast a shadow—ushering in a dark side that demands vigilant attention.
Adversarial AI emerges as a striking example of this darker facet. As artificial intelligence becomes increasingly intertwined with our daily lives, it’s pivotal to recognize that AI systems aren’t immune to vulnerabilities.
Understanding the potential risks and adopting proactive measures is vital to safeguard the integrity and reliability of AI systems. Here through this blog, we will explore some of the vital aspects connected with these AI attacks. So, let’s begin.
What is Adversarial AI?
Adversarial AI refers to a class of techniques and strategies aimed at subverting or manipulating artificial intelligence (AI) systems. These techniques are used to deceive or trick AI models by introducing carefully crafted inputs, known as adversarial AI examples, into the system. Adversarial AI exploits vulnerabilities in AI models, exposing weaknesses in their decision-making processes.
The primary goal of these attacks is to cause AI systems to make incorrect predictions or classifications by subtly altering input data in ways that are imperceptible to humans but highly effective in misleading AI models. These attacks can occur in various domains where AI is utilized, including image recognition, natural language processing, autonomous vehicles, and more.
Impacts of Adversarial Attacks Within Different Sectors
Adversarial AI attacks in today’s digital landscape have far-reaching implications across diverse businesses and industries. These attacks, often stealthy and sophisticated, target vulnerabilities in AI systems, posing significant risks and impact:
Financial Services
Risk of Financial Loss: Adversarial attacks targeting AI-driven algorithms used for trading or investment decisions can lead to erroneous outcomes, causing financial losses or market disruptions.
Fraudulent Activities: Manipulations in automated underwriting systems can result in fraudulent approvals or denials, impacting credit decisions and financial integrity.
Healthcare
Misdiagnosis and Treatment Errors: Adversarial AI attacks on diagnostic systems might lead to misinterpretations of medical images or patient records, potentially leading to incorrect diagnoses or treatment plans.
Patient Data Vulnerability: Breaches in AI systems handling patient records or medical data can compromise confidentiality and patient trust.
Retail and E-commerce
Supply Chain Disruptions: Manipulating AI systems used for inventory management or supply chain optimization can cause errors or delays, affecting product availability and delivery timelines.
Customer Service Interruptions: Attacks on AI-driven customer support systems can lead to service disruptions or incorrect assistance, impacting customer experience.
Autonomous Vehicles and Transportation
Safety Risks: Adversarial manipulations in AI systems guiding autonomous vehicles can pose safety risks, leading to accidents or malfunctioning during critical situations.
Trust and Adoption Challenges: Successful attacks can erode public trust in autonomous technology, affecting the widespread adoption of these innovations.
Cybersecurity and IT Services
Compromised Security Measures: Attacks targeting AI-driven cybersecurity enterprise solutions can lead to vulnerabilities or blind spots, allowing intrusions or breaches.
Loss of Credibility: Breaches in security systems can significantly damage the credibility of cybersecurity firms, impacting client trust.
Types of Adversarial Attacks
Adversarial attacks encompass different types that can significantly impact your business catering to various sectors. It can lead to financial losses, compromised data integrity, operational disruptions, and reputational damage. Here’s an exploration of the types of adversarial attacks on AI and their potential harm to your business:
Evasion Attacks
Adversarial Examples: Crafting subtle modifications to input data, such as images or text, to deceive AI systems. For instance, manipulating product images on an e-commerce platform could mislead image recognition systems, impacting product categorization or search results.
Feature Manipulation: Altering specific features within the data to confuse AI models, potentially leading to incorrect predictions. In financial services, manipulations in credit scoring models could result in flawed risk assessments.
Poisoning Attacks
Data Poisoning: Imagine that you leverage best AI development services, and the solution is being injected with malicious or biased data into training sets. In sectors like healthcare, injecting false medical records into patient databases could impact diagnostic AI systems, leading to erroneous treatment recommendations.
Backdoor Attacks: Inserting hidden patterns or triggers during model training that, when encountered later, cause the system to produce predetermined outputs. This could compromise security systems or authentication processes.
Model Inversion Attacks
Reverse Engineering: Exploiting model outputs to infer sensitive information about the training data. In marketing or customer profiling, adversaries might attempt to deduce proprietary algorithms by analyzing model outputs.
Transferability Attacks
Black-box Attacks: Crafting adversarial AI examples on one AI -driven model and leveraging these to deceive a different but similar model. In sectors reliant on proprietary algorithms, this could compromise intellectual property if models are easily transferable.
Membership Inference Attacks
Membership Inference: Attempting to determine if specific data points were part of the training dataset used to build the AI model. This could reveal sensitive information about clientele, potentially violating privacy regulations.
Physical World Attacks
Adversarial Objects: Manipulating physical objects to deceive AI vision systems. For instance, if your business uses AI-powered surveillance systems, adversaries might alter physical objects to bypass security measures.
Defense Strategies Against Adversarial AI Attacks
Safeguarding your business against adversarial AI attacks involves deploying effective defense strategies tailored to your AI systems. Here’s a rundown on how you can use these strategies:
Robust Model Training
Quality Data Matters: Start by ensuring your training data is diverse, clean, and reliable. High-quality data helps your AI models learn more effectively and reduces susceptibility to manipulations.
Continuous Updates: Regularly update and retrain your models using the latest data. This helps them adapt to evolving threats and strengthens their resilience against potential attacks.
Complexity is Key: Employ sophisticated and intricate model architectures. More complex models often present greater challenges for attackers due to their intricate decision-making processes.
Adversarial Training Techniques
Teach Your Models to Resist: Integrate adversarial AI examples during the training phase. By exposing your models to potential attacks during training, they learn to recognize and resist these manipulations in real-world scenarios.
Diverse Attack Simulations: Generate various adversarial examples during training to broaden your model’s exposure. This diverse training helps protect your system against a wider range of possible attacks.
Ensembling and Model Diversity
Strength in Numbers: Leverage ensemble methods by combining different models. Its diversified approach makes it more challenging for adversaries to exploit common weaknesses across models.
Mix It Up: Use diverse model architectures or training techniques. The more varied your models are, the more difficult it becomes for attackers to find a single vulnerability to exploit.
Model Monitoring and Anomaly Detection
Stay Vigilant: You can choose to leverage the digital transformation services to implement continuous monitoring systems to keep an eye on your model’s behavior. Any unusual patterns or deviations could be indicative of adversarial activities.
Immediate Response: Set up systems for prompt anomaly detection. If anything, suspicious is detected, ensure swift action to investigate and mitigate potential threats.
Looking Ahead: Future Trends for Adversarial AI Defense
Staying ahead in this dynamic landscape requires a holistic and proactive defense strategy that anticipates and evolves with the evolving threat landscape. So, these future trends in adversarial AI defense emphasize the need for innovative, adaptive, and collaborative approaches to fortify AI systems against increasingly sophisticated adversarial threats.
Explainable AI (XAI)
Interpretable Models: Future defense mechanisms will focus on developing AI models that not only perform well but also offer transparency in their decision-making process. This enhances the ability to detect and mitigate adversarial attacks effectively.
Adaptive and Dynamic Defenses
Real-time Adaptation: Anticipating and countering adversarial threats in real-time through AI systems that dynamically adjust and adapt their defenses as attack methodologies evolve.
Generative Adversarial Networks (GANs) for Defense
GANs for Protection: Using GANs not only for adversarial attacks but also as a defense mechanism. It involves generating adversarial examples to fortify AI models against similar attacks. You can leverage generative AI services from the experts and have a secure system against these attacks.
Secure Federated Learning
Distributed and Secure Learning: Leveraging federated learning techniques with enhanced security measures to train models across distributed devices while maintaining data privacy and robustness against adversarial attacks.
Meta-Learning and Few-Shot Learning
Rapid Adaptation: Utilizing meta-learning and few-shot learning techniques to enable AI systems to quickly adapt and learn from minimal data, enhancing their resilience against unseen adversarial attacks.
Certified Robustness and Formal Verification
Mathematically Verified Models: Development of provably robust AI models through formal verification methods, certifying their resilience against specific types of adversarial attacks within defined constraints.
AI Governance and Ethical Frameworks
Ethical Guidelines: Implementing stricter governance and ethical frameworks for AI development. In order to ensure adherence to ethical standards, fairness, and accountability in countering adversarial threats, leveraging the cybersecurity consulting services from the experts.
How Can Matellio Help You in Defeating Adversarial AI?
After scrolling through the impacts, and strategies to protect your business from adversarial AI attacks, you would now agree that a partner is needed. You need experts providing your technology consulting services so that you make the right and secure choices.
This is where you will find Matellio trustworthy!
- We have a team of experts, specialized in crafting customized defense mechanisms tailored to your specific business needs and AI infrastructure. They can design robust security solutions to mitigate adversarial threats effectively.
- Matellio can improve your AI models’ resilience against attacks by integrating adversarial training techniques and fortifying models with enhanced robustness.
- We implement secure infrastructure and data handling practices, safeguarding your sensitive information from potential adversarial attacks.
So we are not just any software development company, rather we are the technology partner that you need by your side to keep your business secure with digital assets.
If you have any doubts or wish to consult our experts for your project idea please feel free to connect with us by filling out this form.
Frequently Asked Questions (FAQs)
What is Adversarial AI?
Adversarial AI refers to the exploitation of vulnerabilities in artificial intelligence (AI) models or systems by intentionally introducing subtle, often imperceptible, modifications to input data. These manipulations are crafted to deceive AI algorithms, leading to incorrect or unintended outputs. Adversarial AI attacks are designed to compromise the reliability, accuracy, or integrity of AI models, posing significant threats across various domains where AI is employed.
How can Adversarial AI Attacks be Defended Against?
Defending against adversarial AI attacks requires a multi-faceted approach incorporating various strategies:
Robust Model Training: Use diverse and high-quality training data, and continuously update and retrain AI models to enhance their resilience against attacks.
Adversarial Training: Incorporate adversarial examples during model training to familiarize AI systems with potential attacks.
Model Monitoring: Implement continuous monitoring to detect anomalies or deviations in the model's behavior.
Ensembling and Diversity: Employ ensemble methods and diverse model architectures to make attacks more challenging.
Explainable AI (XAI): Implement transparency in AI models to understand decision-making processes and detect adversarial manipulations.
Dynamic Defenses: Develop defenses that can adapt in real-time to evolving attack methodologies.
Human-in-the-Loop Approaches: Integrate human expertise to validate critical decisions and counter adversarial activities effectively.
How do We Keep AI safe from Adversaries?
To ensure AI safety from adversaries:
Regular Updates: Continuously update and patch AI systems to address known vulnerabilities and protect against new threats.
Secure Development: Follow secure coding practices and incorporate robust security measures during AI system development.
Data Privacy: Safeguard sensitive data used in AI systems through encryption and secure data handling practices.
Ethical AI Practices: Adhere to ethical guidelines and compliance standards to ensure responsible AI development and deployment.
Training and Awareness: Educate employees on cybersecurity best practices and raise awareness about potential adversarial threats to enhance vigilance.
What is Adversarial Search in AI?
Adversarial search in AI refers to a search algorithm used in competitive settings, notably in games where two or more players compete. It involves the exploration of potential moves or actions considering an opponent's strategy or counteractions. Adversarial search algorithms, like minimax or alpha-beta pruning, aim to find optimal strategies while anticipating and countering an opponent's moves to maximize the chances of success in competitive scenarios.
What are adversarial AI examples?
Adversarial AI encompasses various examples where AI systems are manipulated or deceived through subtle alterations in input data. Here are some instances of adversarial AI examples:
Image Classification Manipulation:
Altering or adding imperceptible noise to images to mislead AI models. For instance, adding subtle perturbations to stop signs, causing AI systems to misclassify them as other road signs.
Spam and Malware Evasion:
Crafting emails or malicious code that bypass spam filters or antivirus systems by subtly altering content or code to evade detection.
Voice Command Manipulation:
Crafting audio inputs with imperceptible noise or alterations that cause virtual assistants or speech recognition systems to misinterpret commands or execute unintended actions.
Adversarial Objects in Visual Recognition:
Creating physical objects (e.g., eyeglasses frames or stickers) with slight modifications to deceive AI-based visual recognition systems, causing misclassification or recognition errors.
Financial Fraud Detection Evasion:
Manipulating financial data or transactions with slight alterations to deceive AI-based fraud detection systems, leading to false positives or negatives in fraud identification.
Autonomous Vehicle Misdirection
Altering road markings or traffic signs in ways imperceptible to humans but confusing to AI-driven autonomous vehicles, potentially causing navigation errors.
Medical Image Misinterpretation:
Introducing imperceptible alterations in medical images or patient records to deceive AI diagnostic systems, leading to incorrect diagnoses or treatment recommendations.
These examples demonstrate how subtle manipulations in data can lead AI systems to make incorrect or unintended decisions, highlighting the vulnerabilities and potential risks associated with adversarial AI attacks.