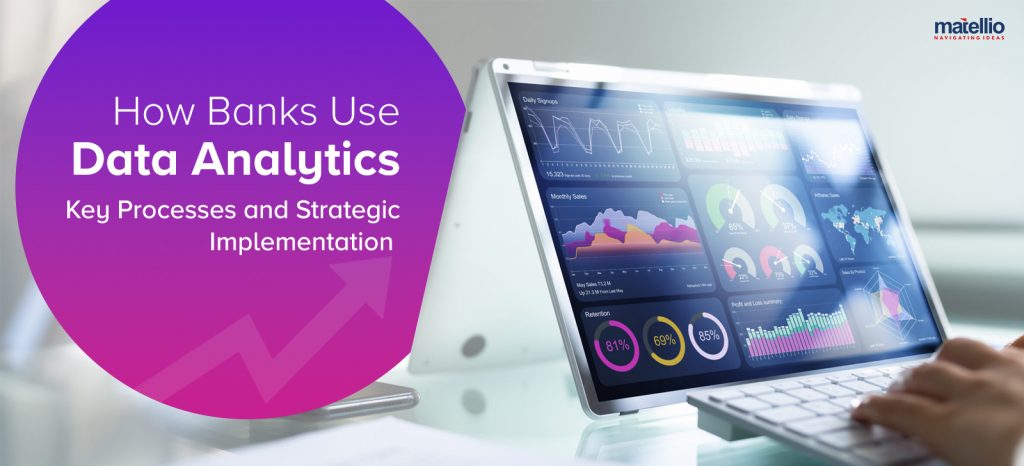
In the rapidly evolving banking industry, the importance of data analytics in banking has reached unprecedented levels. With the exponential growth of data and advancements in technology, banks are now able to harness vast amounts of information to gain actionable insights.
These insights are pivotal for enhancing customer experiences, predicting and mitigating risks, and ensuring stringent regulatory compliance. Data analytics is no longer just a supplementary tool; it has become the backbone of strategic decision-making and operational efficiency in modern banking.
This blog delves into how banks use data analytics, focusing on the strategic implementation processes and key aspects that banks must consider to fully leverage this transformative tool. We will explore the comprehensive steps and strategies that are reshaping the banking landscape through data analytics.
The Role of Data Analytics in Banking
How banks use data analytics significantly impacts their decision-making processes and overall operational efficiency. By analyzing vast amounts of data, banks can make informed decisions that drive growth and enhance their service offerings. Here are several key areas where the use of data analytics in banking plays a crucial role:
Personalized Interactions
Banks can use data analytics to understand individual customer preferences and behaviors, allowing for highly personalized services and product recommendations. This personalization increases customer satisfaction and loyalty.
Real-Time Monitoring
Advanced analytics enable banks to monitor transactions in real-time, identifying suspicious activities as they occur. This immediate detection allows banks to prevent fraudulent transactions before they are completed.
Credit Risk Assessment
By analyzing customer credit history, transaction behavior, and other relevant data, banks can build more accurate credit scoring models. This improves the ability to assess credit risk and make informed lending decisions.
Process Optimization
Data analytics can identify inefficiencies in banking operations, such as bottlenecks in loan processing or customer service. By streamlining these processes, banks can reduce costs and improve service delivery.
Risk Management Frameworks
Analytics can help in developing robust risk management frameworks that comply with regulatory standards, ensuring that all potential risks are identified and managed effectively.
Behavioral Insights
By analyzing customer transaction data and engagement patterns, banks can gain deep insights into customer behavior. It helps in creating targeted marketing campaigns and personalized product offerings.
Steps to Implement Data Analytics in Banks
Define Objectives
The first step in how banks use data analytics is to define clear objectives. Banks must determine what they aim to achieve with data analytics, such as improving customer experience, enhancing risk management, or increasing operational efficiency. Clearly defined objectives guide the data analytics strategy and ensure alignment with the bank’s overall goals.
- Align with Business Strategy: Establish objectives that support the bank’s broader business strategy. For example, if the goal is to enhance customer satisfaction, the focus should be on customer behavior analytics and personalization.
- Set Measurable Targets: Define specific, measurable targets, such as reducing fraud incidents by 20% or increasing loan approval efficiency by 15%. These targets provide a benchmark for success and help track progress.
Data Collection
Next, banks need to gather data from various sources. This includes transaction records, customer feedback, social media interactions, and market reports.
- Internal Data Sources: Collect data from internal systems such as CRM, transaction logs, customer service interactions, and financial reports.
- External Data Sources: Leverage external data sources, including social media platforms, market research reports, economic indicators, and third-party data providers.
- Data Integration: Integrate data from these various sources to create a unified dataset that provides a holistic view of customer behavior and operational performance.
Data Cleaning and Preparation
Collected data must be cleaned and organized to ensure accuracy. This involves removing duplicates, handling missing values, and standardizing formats. Proper data preparation is crucial for reliable analytics outcomes.
- Data Validation: Validate the data to ensure accuracy and consistency. This step involves checking for errors, inconsistencies, and outliers.
- Data Enrichment: Enhance the data by integrating additional relevant information, such as demographic details or market trends, to provide deeper insights.
- Standardization: Convert data into a standardized format to ensure uniformity across the dataset, which is critical for effective analysis. Leveraging the right data consultation services can help with conversion.
Choosing the Right Tools and Technologies
Banks should choose tools that align with their objectives and infrastructure, whether it’s Hadoop for big data processing or advanced software for machine learning.
- Evaluation of Tools: Assess different data analytics tools for their capabilities, scalability, and compatibility with existing systems. Tools may include data warehousing solutions, machine learning platforms, and visualization software.
- Cloud vs. On-Premises: Decide whether to use cloud-based solutions or on-premises infrastructure. Cloud solutions offer scalability and flexibility, while on-premises options provide greater control over data security.
- Integration with Existing Systems: Ensure that the chosen tools can seamlessly integrate with existing IT infrastructure and legacy systems.
Note: The images above showcase the basic representation of the dashboard and can be changed as per the requirements.
Building Analytical Models
Developing analytical models is a core part of how data analytics is used in banking. Predictive models, customer segmentation, and risk assessment tools are built to analyze the collected data and provide actionable insights.
- Model Development: Use statistical and machine learning techniques to develop models that can predict future trends, segment customers, and assess risks.
- Model Testing and Validation: Test the models with historical data to validate their accuracy and reliability. Adjust the models as needed to improve performance.
- Customization: Tailor models to the bank’s specific needs and objectives, ensuring they address unique challenges and opportunities.
Implementing the Analytics Solutions
Integrating these models into the bank’s systems and processes ensures that insights are actionable. This step involves embedding analytics into daily operations, from customer service to fraud detection.
- System Integration: Integrate analytical models into the bank’s IT systems, ensuring seamless data flow and accessibility.
- Automation: Automate routine tasks and decision-making processes using analytics, as it frees staff to focus on more complex issues.
- User Training: Train employees on how to use new analytics tools and interpret the results. This ensures that staff can leverage the insights effectively in their roles.
Monitoring and Optimization
Continuous monitoring of data analytics models is necessary to maintain their effectiveness. Banks should regularly review model performance and adjust as needed to adapt to new data and evolving market conditions. This step is crucial in how banks use data analytics to stay agile and responsive in a dynamic environment.
- Performance Tracking: Use key performance indicators (KPIs) to monitor the effectiveness of analytics initiatives. This helps identify areas that require improvement.
- Regular Updates: Update models regularly with new data to ensure they remain relevant and accurate. This includes retraining machine learning models with the latest data.
- Feedback Loop: Establish a feedback loop where insights from analytics are used to inform business strategies, and business outcomes are fed back into the analytics process for continuous improvement.
Want to Harness the Power of Data Analytics and Enhance Your Operations?
Key Factors to Consider
Data Privacy and Security
Ensuring the protection of customer data is very important. It is why the banks must implement robust security measures to prevent data breaches.
- Encryption: Use encryption to protect data at rest and in transit, ensuring that unauthorized parties cannot access sensitive information.
- Access Controls: Implement strict access controls to limit who can access sensitive data. Role-based access can help in segregating data access based on the user’s role.
- Incident Response Plans: Develop and maintain incident response plans to quickly address and mitigate the impact of any data breaches or security incidents.
Regulatory Compliance
Adhering to banking regulations and standards is essential. Accurate data reporting and compliance with regulations help avoid legal issues.
- Compliance Tools: Utilize advanced tools that automate compliance reporting and monitor regulatory changes. These tools can help in ensuring that the bank remains compliant with evolving regulations.
- Regular Training: Ensure staff are regularly trained in compliance requirements and updates. Continuous education helps employees stay informed about regulatory changes and best practices.
- Documentation and Reporting: Maintain thorough documentation of compliance processes and reporting procedures to demonstrate adherence to regulatory requirements during audits.
Data Quality
Maintaining high-quality data is critical for accurate analysis. Poor data quality can lead to incorrect insights and decisions.
- Data Governance: Establish strong data governance policies to maintain data integrity. This includes defining data ownership, establishing data quality standards, and implementing data stewardship roles.
- Data Cleansing: Implement routine data cleansing processes to remove inaccuracies and inconsistencies. Regular data audits can help identify and rectify data quality issues.
- Metadata Management: Use metadata management practices to ensure data is well-documented and easily traceable, improving data accuracy and usability.
Data Integration
Integrating data from various sources for a holistic view can be complex. Effective data integration is essential for leveraging insights from diverse data sets and ensuring accurate, comprehensive analytics.
- Unified Data Platform: Use a unified data platform to consolidate data from different sources, ensuring a seamless flow of information across the organization.
- Data Integration Tools: Employ advanced data integration tools that facilitate seamless data merging and ensure consistency. These tools can help automate the process of data extraction, transformation, and loading (ETL). Partnering with a fintech software development company can provide customized integration solutions tailored to the bank’s specific needs, enhancing efficiency and reliability.
- Interoperability Standards: Adopt interoperability standards to ensure that data from different systems and platforms can be integrated smoothly. Standardizing data formats and protocols helps in achieving seamless integration and improves data accessibility across the organization.
Scalability
As data grows, the ability to scale analytics solutions is crucial. Effective scalability ensures that the use of data analytics in banking can continuously evolve to meet increasing demands and data complexity.
- Cloud Solutions: Leverage cloud-based analytics platforms that offer scalability, flexibility, and cost-effectiveness.
- Modular Architectures: Implement modular system architectures that can grow with your data needs, allowing for incremental upgrades and expansions.
- Performance Optimization: Regularly monitor and optimize system performance to ensure that analytics solutions can handle increasing data loads efficiently.
Use Cases of Data Analytics in Banks
Fraud Detection
Data analytics is important in banking because it identifies suspicious activities and transactions to prevent fraud.
- Real-Time Monitoring: Implement systems that monitor transactions in real time to detect fraud early. Real-time analytics can help identify anomalies and flag suspicious activities instantly.
- Behavioral Analytics: Analyze customer behavior to identify deviations from normal patterns that may indicate fraud. Behavioral analytics can provide deeper insights into potential fraud risks.
Customer Segmentation
By grouping customers based on behaviors and preferences, banks can target marketing efforts more effectively.
- Behavioral Analysis: Analyze spending habits and transaction history to segment customers into distinct groups. This helps in creating targeted marketing campaigns.
- Lifecycle Segmentation: Segment customers based on their lifecycle stages, such as new customers, loyal customers, or at-risk customers, to tailor retention strategies accordingly.
Risk Management
Assessing credit risks and predicting loan defaults through data analytics helps banks manage and mitigate risks. By incorporating AI integration services, banks can enhance their risk management strategies with more advanced and accurate tools.
- Predictive Analytics: Use predictive models to forecast loan default risks and take proactive measures to mitigate them. This includes adjusting loan terms and interest rates based on risk assessments.
- Stress Testing: Conduct stress tests using predictive analytics to evaluate the bank’s resilience to adverse economic scenarios and identify potential vulnerabilities.
Personalized Banking
For enhancing the customer experience offering tailored products and services based on customer data is vital.
- Recommendation Engines: Use data to suggest products and services that meet individual customer needs, such as personalized loan offers or investment recommendations.
- Customer Journey Mapping: Analyze customer interactions across various touchpoints to create personalized experiences and improve customer satisfaction.
Customer Lifetime Value (CLV) Analysis
Calculate the expected value generated from a customer over their entire relationship with the bank.
- Retention Strategies: Develop strategies to increase customer retention based on CLV insights, such as loyalty programs and targeted offers.
- Targeted Campaigns: Focus marketing efforts on high-value customers to maximize returns on investment.
Operational Efficiency
Streamlining internal processes and reducing operational costs through data-driven decisions improves overall efficiency. Leveraging digital transformation services can significantly enhance these efforts, enabling banks to modernize their operations and optimize performance.
- Process Optimization: Identify inefficiencies and optimize workflows using data analytics. This can include automating routine tasks and improving process efficiency.
- Resource Allocation: Use data to better allocate resources and manage workloads, ensuring that the right resources are available where they are needed most.
Wish to Implement Data Analytics in Your Various Banking Operations?
Future Trends in Data Analytics for Banking
Adoption of AI and Machine Learning
Banks are increasingly adopting AI and machine learning for more advanced analytics capabilities. The use of AI in banking is transforming the way financial institutions operate, providing deeper insights and enhancing efficiency.
- Advanced Algorithms: Use deep learning and neural networks for complex data analysis, enabling more accurate predictions and insights.
- Automation: Automate routine tasks using AI to improve efficiency and accuracy, such as automated customer service through chatbots and virtual assistants.
Increased Focus on Real-Time Data Analytics
The ability to analyze data in real time is becoming more important for immediate decision-making.
- Instant Insights: Provide real-time insights to support instant decision-making, such as real-time fraud detection and risk assessment.
- Event-Driven Analytics: Use real-time data to respond to events as they happen, improving agility and responsiveness.
The Growing Importance of Big Data in Banking
Big data continues to play a critical role in how banks use data analytics, providing deeper insights and more comprehensive analyses.
- Data Lakes: Create data lakes to store vast amounts of structured and unstructured data, enabling advanced analytics and data exploration.
- Advanced Analytics: Use big data analytics to uncover hidden patterns and trends, driving strategic decision-making and innovation.
Cloud Computing and Analytics
Cloud computing integration with data analytics provides scalable and flexible solutions for banks. Leveraging cloud integration services allows banks to enhance their data analytics capabilities while maintaining cost efficiency and scalability.
- Scalability: Cloud-based analytics platforms can scale to handle large volumes of data and complex analytics workloads.
- Cost Efficiency: Cloud solutions offer cost-effective alternatives to traditional on-premises infrastructure, reducing capital expenditure and operational costs.
Enhanced Customer Experience through Omni-Channel Analytics
Banks are focusing on providing a seamless customer experience across multiple channels to meet the diverse needs of modern customers. Omni-channel analytics helps integrate data from various customer touchpoints, ensuring a consistent and personalized experience.
- Omni-Channel Insights: Integrate data from various customer interaction channels, which include online banking, mobile apps, and physical branches, to provide a unified view of the customer experience.
- Personalized Interactions: Use omni-channel analytics to deliver personalized interactions and consistent experiences across all touchpoints.
Ethical AI and Analytics
Ensuring the ethical use of AI and data analytics in banking is crucial for maintaining trust and fairness. As banks increasingly rely on AI for decision-making, it is significant to address potential biases and ensure transparency in AI models and processes.
- Bias Mitigation: Implement strategies to identify and reduce bias in AI models, ensuring fair and equitable outcomes.
- Ethical Guidelines: Develop and adhere to ethical guidelines for data usage, promoting transparency and accountability in AI and analytics practices.
Hyper-Personalization
Utilizing AI to deliver highly personalized customer experiences is a cutting-edge approach to how banks use data analytics to enhance customer satisfaction and engagement.
- Dynamic Personalization: Use real-time data to personalize customer interactions dynamically, such as personalized product recommendations and offers.
- Customer Micro-Segmentation: Create highly detailed customer segments for targeted marketing, improving relevance and engagement.
Consult Experts to Explore How These Trends Can Help Your Business Grow!
How Can Matellio Guide Banks On Using Data Analytics?
By understanding how banks use data analytics and following a strategic implementation process, banks can unlock significant value and maintain a competitive edge in the industry. You must prioritize investing in data analytics to leverage its full potential and ensure sustainable growth.
If you’re seeking a reliable partner for this transformative journey, Matellio is here to support you every step of the way!
- Our team offers strategic insights and customized solutions tailored to your bank’s specific needs. We collaborate closely with you to understand your objectives and develop a comprehensive data analytics strategy that aligns with your business goals.
- Our approach ensures that the integration of data analytics enhances user experience, streamlines workflows, and supports organizational growth.
- Our team provides 24/7 technical assistance, regular updates, and proactive monitoring to ensure your data analytics system operates efficiently and remains current with technological advancements.
- With our comprehensive technology consulting services, we help you seamlessly integrate advanced analytics into your existing systems, driving significant improvements in performance and scalability.
Feel free to contact us today by filling out the form to learn more about how we can empower your business with data analytics.
FAQs
Q1. Can data analytics in banking integrate with existing systems?
Yes, we specialize in creating hybrid solutions that seamlessly integrate data analytics with both legacy systems and modern architectures. This approach allows you to leverage the benefits of data analytics in banking while preserving your current IT investments.
Q2. What are the costs associated with data analytics in banking services?
The costs for data analytics in banking services vary based on factors such as project complexity, technology requirements, customization needs, and integration specifications. At Matellio, we provide transparent cost estimates tailored to your specific organizational needs, ensuring a solution that aligns with your budget and strategic objectives.
Q3. What support and maintenance do you offer for data analytics in banking?
Our team provides comprehensive support throughout the integration process, including 24/7 technical assistance, regular updates, performance optimization, and proactive monitoring. Our goal is to ensure that your data analytics system in banking operates smoothly, remains up to date with technological advancements, and delivers reliable and actionable insights.
Q4. Can data analytics in banking solutions be customized to meet specific organizational needs?
Absolutely. We specialize in tailoring integrated solutions to meet the unique requirements of your organization. Whether you require specific functionalities, integration with existing systems, or customized workflows, our team collaborates closely with you to design and implement a solution that enhances operational efficiency and supports your strategic business goals.
Q5. How is data analytics used in banking?
Data analytics in banking is used to enhance various aspects of operations, including customer experience, risk management, fraud detection, and regulatory compliance. By analyzing large volumes of data, banks can gain insights into customer behavior, predict risks, personalize services, and streamline processes, ultimately driving better decision-making and efficiency.