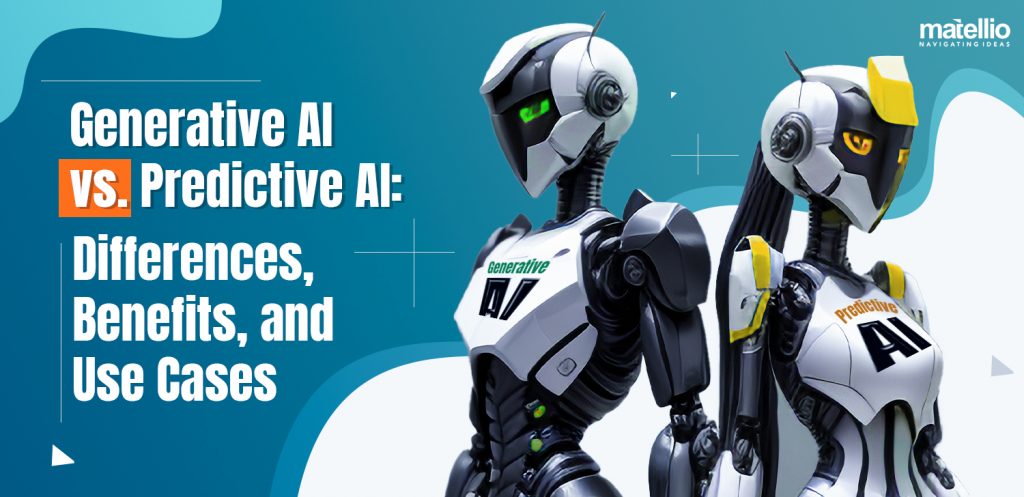
Are you confused between generative AI vs. predictive AI for your next enterprise solutions development? Well, why not; after all, both these branches of AI have gained significant attention in less time. While sharing a common goal of leveraging AI for insights and decision-making, these two approaches diverge in their methodologies and outcomes.
So, which is better generative AI vs. predictive AI, for your business? Well, that calls for exploring their advantages, disadvantages, and use cases, and that’s what our today’s blog post is all about!
In this blog, we will understand and compare generative vs. predictive AI. We will delve into the core principles of each approach, explore their respective use cases, and unravel their potential for the future.
Whether you’re a data enthusiast, a business leader, or simply curious about the possibilities of AI, join us as we unravel the fascinating world of generative AI vs. predictive AI.
Why Do You Need to Invest in AI Development Services?
Before proceeding further with the choice of generative AI vs. predictive AI, many of you might have this question why choose AI development services for business automation? Although AI has become an essential component, a few companies are still in the dilemma of whether to choose AI for software development!
So, here are some reasons that will help you make a better decision:
Image: Why Investing in AI Development Services a Profitable Move
- The global market for AI development services will reach $308 billion by 2026.
- The AI adoption rate will be more than 60%, with the highest adoption of AI digital solutions in healthcare, finance, and retail.
- By 2025, generative AI will be responsible for 10% of all data curated.
- 84% of people use one or more than one AI-powered application or services.
- 85% of all customer interactions in enterprises, SMEs, and even startups will be handled by custom chatbots.
- 97% of mobile users leverage AI-based voice assistants for different functions.
- 86% of CEOs agree that AI and business go hand-in-hand since AI is mainstream technology in their offices.
As evident from the stats above, AI has become essential to running a successful business. Whether finance, healthcare, retail, or even real estate or logistics, global companies across various industries are leveraging AI development services to boost growth, innovation, and profitability. So, why should you lag?
Connect with our experts over a free consultation call to learn how AI development services can accelerate your digital transformation journey!
Now, coming to the question of choosing between generative AI vs. predictive AI, let us explore their core definitions, benefits, limitations, and use cases to help you make a better choice.
What is Predictive AI?
Predictive AI, also known as predictive modeling, is powerful artificial intelligence technique businesses use to make highly accurate predictions about things such as inventory levels, demand in the future, consumer preferences, etc.
Companies from all industries use predictive AI to analyze historical data and make rapid predictions, projections, or forecasts about the future.
Machine learning and statistical modeling combine to predict future outcomes or requests. Predictive AI models can identify anomalies, identify consumer buying and purchasing trends, and uncover future consequences.
By providing predictive AI models with data and training them to recognize nuances and relationships, companies can anticipate trends, identify anomalies, detect fraud, prevent equipment failures, optimize marketing campaigns, customize user experiences, and more.
Predictive AI techniques sometimes help companies reduce operational risks by adjusting their forecasts over time as new data becomes available.
Numerous industries, most notably healthcare, banking, retail, supply chain, manufacturing, and logistics, employ predictive AI to gain a competitive edge using data-driven insights and better decision-making.
Read More: How is AI Impacting Predictive Analysis?
How Does Predictive AI Work?
Machine learning models and data analysis strategies are used in predictive AI. In order to develop a clear connection and forecast future events, it first collects and analyzes past business data before identifying common patterns and relationships between various data sets.
Here’s how predictive AI works for generating the most suitable predictions and business outcomes:
Data Collection
The first step in predictive AI is data organization and gathering because machine learning algorithms need to analyze data. Predictive AI gathers relevant information from reliable sources such as historical data, consumer data, trends in the market, sensor data, etc.
Data Preprocessing
Data preprocessing is the next important step in the work of predictive AI models. After the models have collected data, the data goes through preprocessing, which is sorted and cleaned for analysis. The preprocessing process often includes missing values, data standardization, and outliers’ removal.
Feature and Algorithm Selection
In the third stage, the predictive AI models select the most suitable features and algorithms. The selection of features and algorithms has a direct effect on the performance and accuracy of the model. If you select data from trusted sources, you will get accurate predictions; else, not! Some common machine-learning methods are linear regression, decision trees, random forest, support vector machine, neural network, etc.
Creating Training Data
Training data is divided into two categories in the selected engineered dataset: testing data and validation data. Training data gives the predictive AI model input features and their target values (the predicted value). Training data is used to build the model.
Model Selection and Training
The chosen model is trained on decided training data. During training, the model learns the relationships and patterns in the data and makes predictions. During the training, the model changes its internal parameters to reduce the difference between predicted and actual values.
Model Testing and Evaluation
After training, the predictive AI model is tested/validated using the training data. Then, you can evaluate the model’s performance and generalizability to new data.
Future Prognoses and Results
The next step for predictive AI algorithms is to make predictions and predict future outcomes. After training and evaluation, the model will be ready to make predictions based on the new, unexpected data. The model uses the input data to make predictions or estimates of the probability of the target variable.
A crucial thing to remember here is that the accuracy of the predictions made by the AI models depends on the quality and accuracy of the data sets. Hence, you should always leverage reliable data consulting services to ensure that the source of the data collection is reliable and there is sufficient data for model learning.
What are the Benefits of Predictive AI?
So, we explored the basic definition and working of predictive AI. But the main question is, what benefits does it offer? Why are companies across the globe leveraging predictive AI services to boost their business growth? Let’s discover the amazing benefits of predictive AI:
Accuracy in Predicting Future Trends and Customer Requirements
The primary benefit of predictive AI is its ability to accurately predict future trends and customer needs. We all know that predictive AI algorithms analyze past data to identify trends and correlations across different data sets. Therefore, you can use this data to forecast market trends and client requirements. This will eventually allow you to adjust your marketing strategies and corporate policies so that you can deliver products and services that meet your clients’ needs.
Improved Demand Planning and Forecasting
One of the biggest benefits of predictive AI is that it helps with demand planning and forecasting. Predictive AI models use machine learning and data analysis techniques to analyze past sales data, market dynamics, customer behavior, and other relevant factors. The predictions for product demand are made based on the processed data.
For instance, you can see which products are the most popular with your clients and which ones do not need to be reordered to save money. This benefit allows you to effectively manage your inventory levels, reducing expenses, eliminating wasteful spending, and increasing supply chain efficiency.
Better Customer Experiences
Personalization is the key to success in today’s competitive business era. That’s where predictive AI comes into play! Whether you need to find the chances of success of your new product or your target market’s preferred choices, predictive AI will give you the data you need. Based on past customer interactions with your business, this AI technique allows you to deliver targeted recommendations, personalized marketing messages, and unique product offerings.
Fraud and Cybersecurity Threats are Eliminated
This benefit will absolutely blow your head if you believe that predictive AI is solely about planning and forecasting future demand. Some businesses employ predictive AI to get rid of hazards and fraud. Data from transaction logs, user activity patterns, network logs, and other prominent data sources are analyzed by predictive AI models. Then it looks for anomalies or strange patterns linked to fraud or other cybersecurity concerns. In this manner, you can prevent fraud or security risks by taking preventative measures.
Making Decisions Based on Data
Last but not least, using predictive AI approaches in your company’s operations has the benefit of enabling data-supported decisions. CIOs and CXOs can make use of the comprehensive business insights provided by predictive AI to make wise decisions. In order to provide a deeper insight into market trends, client preferences, and other important elements, smart models evaluate historical data. This enables firms to decide with confidence on a variety of issues, including product creation, pricing tactics, marketing initiatives, resource allocation, and process improvement.
What are the Limitations of Predictive AI?
Predictive AI is, without a doubt, the area of artificial intelligence that offers the most promise. Predictive AI does, however, have two sides, just like every coin. Predictive AI falls short in the following areas:
Erroneous Predictions
The accuracy of predictions is the main area where predictive AI might fall short. We are all aware that effective predictions made by predictive AI rely greatly on the reliability and accessibility of data. However, the performance of the prediction model may suffer if the source of the data is unreliable or if the data is inaccurate, noisy, or biased.
Privacy Issues with Data
The privacy hazards associated with using personal or company data are yet another significant drawback of predictive AI. There is some chance that the process of gathering, storing, and analyzing the data will violate people’s right to privacy and ethical considerations.
Complexity of Interpretation
Last but not least, prediction models like deep learning neural networks are incredibly sophisticated and challenging to understand. It is difficult to grasp how the AI model generates its predictions because of this lack of interpretability. Interpretability is essential for building confidence and guaranteeing openness in decision-making processes in delicate industries like healthcare or finance.
What is Generative AI?
The field of generative AI is yet another robust branch of artificial intelligence that is today widely used across various industries. It is utilized in numerous industries for data augmentation, content creation, image creation, and music creation. With the help of generative AI, models are created that generate fresh content based on patterns discovered in the training data set. The generative AI models produce slightly different copies of the information by combining deep learning and machine learning methods.
The most promising generative AI outcomes are applied to enhance consumer experiences across a wide range of platforms and sectors. Using text input, generative AI can produce fresh versions of books, mimic human behavior in customer interactions, and generate music, images, and text. The main advantage of generative AI services lies in the fact that it does not create something that already exists. Instead, it uses a range of different machine learning algorithms to distinguish between the content that it has delivered to the users and the content that already exists in its database.
How Does Generative AI Work?
There are numerous critical steps that go into how generative AI functions. For instance, generative adversarial networks (GANs) are one of the major methods employed in generative AI. It essentially combines a generator network with a discriminator network.
The discriminator network attempts to differentiate between the created samples and the actual samples from the training data, while the generator network creates new examples.
Here’s how the generative AI models work to produce high-quality results:
Data Gathering
First, a dataset of unique items representing the target domain must be put together. For instance, if your goal is to make realistic cat graphics, you need to collect a lot of cat photos. The same holds true for the addition of data, text, and music.
Structure Design
Designing the architecture of the generator and discriminator networks, which, when coupled, constitute GANs (generative adversarial networks), is the next step taken by generative AI models. The discriminator concentrates on telling the difference between the generated samples and the real ones, while the generator employs random noise as input and aims to produce realistic data samples.
GAN Model Training
The GAN model is trained to improve its results in the following stage. The generator in this method generates random samples, which the discriminator subsequently analyzes. Each sample is classified by the discriminator as real or artificial. Both networks gradually boost their performance via backpropagation and tuning methods.
Adversarial Training
It is a procedure that takes place during the training of AI models. With further training, the generator becomes more adept at creating samples that deceive the discriminator. At the same time, the discriminator becomes more adept at correctly identifying the created samples.
Performance Evaluation
The performance of the enhanced GAN model is then assessed using new samples. In this process, you can evaluate the results qualitatively by visually inspecting them, or you can compare metrics like picture quality, similarity to real samples, or domain-specific metrics.
What are the Benefits of Generative AI?
Generative AI is today extensively used across various industries and businesses. Right from content creation and music enhancement to image creation, data representation, and even personalized customer experiences, it offers several benefits that lure companies to adopt generative AI for their businesses.
Better Inspiration and Creativity
The key benefits of generative AI are increased inspiration and creativity. We are all aware that the main responsibility of generative AI models is to produce new material that meets user requirements. For those seeking new and unique designs, artwork, photography, movies, and other forms of creative output, generative AI can therefore be immensely helpful. In fact, it might be a great innovation-spurring tool for companies operating in industries like graphic design, advertising, and entertainment.
Data Improvement
Existing datasets can be enhanced and expanded thanks to generative AI’s ability to create synthetic data that closely mimics real data. This is especially helpful in fields where collecting data is costly, time-consuming, or difficult. Machine learning models can perform better and be more dependable when fed fictitious data.
Improved Customer Experiences
Generative AI allows for the creation of individualized recommendations, information, and experiences. Customer happiness may increase as a result of AI systems making personalized and pertinent suggestions for goods, services, and entertainment based on user data and preferences.
Better Simulation and Training
The ability of generative AI to generate training data and replicate real-world scenarios is another outstanding advantage. Because it can be challenging or expensive to manually collect enormous volumes of training data in these fields, this modeling of real-world scenarios is very helpful in businesses like robots, autonomous vehicles, and virtual reality. Using generative AI, businesses may test how a specific application might function in real-world scenarios.
Innovation and Privacy Protection
Finally, generative AI accelerates medical innovation and research while securing critical data. Generative AI models are widely used in several facets of the healthcare industry, including drug research, disease simulation, and medical image analysis. By creating synthetic medical data, it aids in data anonymization, privacy protection, and the interchange of sensitive medical information.
What are the Limitations of Generative AI?
Although generative AI is extensively used across various industries, this robust AI technology has some limitations. So, what are they, and how can you eliminate them by partnering with an experienced AI development company? Let’s find that out!
Ownership Concerns
We all know that generative AI uses machine learning and deep learning models to analyze the original content and make new content out of it. However, that may raise ethical concerns for some users. We cannot detect which one was the original content. Moreover, the problems of biased or inappropriate content are also a major drawback of generative AI.
Limited Access to Information
Generative AI models work solely on the data provided by the company. Hence, they do not have access to the extra information that may not be fed to them during the training period. That’s why generative AI cannot be used in areas related to research, statistics, etc.
Read More: Generative AI for Business: Explore Use Cases, Industries, And Strategies
Generative AI vs. Predictive AI – What to Use?
So, we are at the most awaited section of our blog post – choosing between generative vs. predictive AI. Obviously, the two major and latest types of artificial intelligence techniques, namely predictive and generative, have the potential to significantly alter company operations. While generative AI uses artificial neural networks and deep learning algorithms to produce new material, predictive AI concentrates on finding patterns in data to forecast future outcomes.
So, would you be better off using one over the other? Well, the answer is no!
Each variety has its own advantages, but the one you choose will rely on the particular requirements or objectives you want to meet. Consider the situation where you are working with a lot of client data. Predictive models might then prove useful in better-comprehending consumer behavior to produce forecasts about their purchasing decisions that are more accurate.
Alternatively, let’s imagine your ambition is to engage in creative work, such as designing products or coming up with advertising. In that case, generative models might be the most useful as, in contrast to normal machine learning techniques, they can generate novel, creative ideas from datasets without heavily relying on precedent examples.
So, to conclude, we can say that choosing between generative AI vs. predictive AI is a difficult process because it demands a deep analysis of your business needs and long-term objectives. But whichever field of AI you choose, it will significantly boost productivity and workflow effectiveness in today’s office!
To find the best match between generative vs. predictive AI for your organization’s needs, however, be sure to get in touch with a reputable and knowledgeable AI development company.
That’s Where Matellio Steps In!
As a leading AI development company, we aim to help you choose the best services and solutions focused on expanding your business. Whether you seek data consulting services, AI-based data analytics services, generative AI development services, or even predictive AI services, we are here to help you.
With more than a decade-long experience, we have been helping businesses across the globe to boost innovation and profitability via AI solutions and services. Whether telecom, real estate, healthcare, retail, logistics, or even finance and education, companies across diverse industries trust us with their AI software development projects.
We have also been recognized by brands like Clutch, Design Rush, and more for our top digital transformation services. So, leverage our expertise and professional services to innovate your business using generative AI or predictive AI.
Connect with our experts over a free consultation call to discuss your business needs and ideas and get a no-obligation quote and the best assistance on choosing between generative AI vs. predictive AI for your business. Fill out our form to book a free 30-min expert consultation.