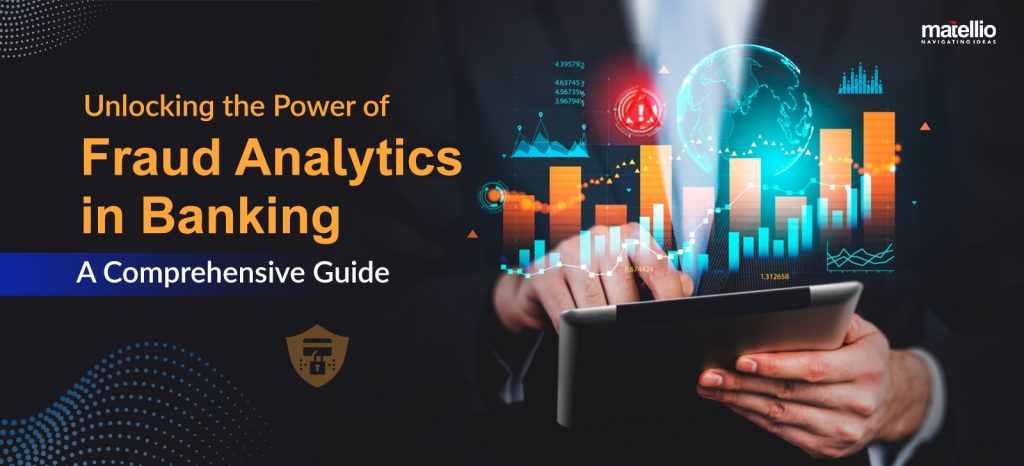
In the ever-evolving financial landscape, banking institutions face a significant challenge: fraud. With the rise of digital banking and financial technology, the complexity and frequency of fraudulent activities have increased. To combat this growing threat, banks need to adopt advanced fraud detection mechanisms. Fraud analytics in banking plays a pivotal role in safeguarding financial institutions, ensuring the integrity of transactions, and maintaining customer trust.
Fraud in banking is not a new phenomenon. Historically, fraud was primarily conducted through physical means, such as check forgery and cash embezzlement. However, as banking operations digitized, fraud tactics evolved. The advent of online banking introduced new vulnerabilities, leading to a surge in cyber fraud and identity theft. In the digital age, fraudulent tactics have become more sophisticated. Cybercriminals use advanced techniques, such as phishing, malware, and social engineering, to exploit vulnerabilities in banking systems. The anonymity provided by digital transactions has made it easier for fraudsters to execute large-scale fraud schemes, posing a significant threat to financial institutions. Fraud analytics in banking relies on a robust framework comprising several core components and methodologies. These elements work together to detect, prevent, and mitigate fraudulent activities effectively. Let’s explore each component in detail: Fraud detection using data analytics in the banking industry offers several key advantages for banks, enhancing their ability to detect and manage fraudulent activities: Sophisticated algorithms identify subtle patterns and anomalies more effectively than manual methods. Real-time monitoring enables immediate action to prevent fraud. Early warning systems and risk scoring help identify potential fraud before it happens, allowing for timely intervention and prioritization of suspicious activities. Ensures compliance with standards like AML (Anti-Money Laundering) and KYC (Know Your Customer) and maintains detailed audit trails. This helps avoid non-compliance penalties and improves regulatory reporting. Protects customer assets and ensures confidentiality of personal information, enhancing trust. Reduces disruptions and improves the customer experience by minimizing account freezes and providing transparent communication. Customized fraud analytics solutions can automate routine tasks, reducing manual intervention and ensuring consistent handling of fraud cases. Optimizes resource use and scales with increasing transaction volumes. Early fraud detection reduces financial losses and minimizes recovery costs. Automates initial investigation stages to lower investigation costs and allocate resources more effectively. Provides deep insights into transaction data and fraud patterns, aiding in strategic planning and operational improvements. Helps in identifying trends and managing risks more effectively. Also Read: The Role of RPA in Fraud Detection and Prevention through Transaction Monitoring Ready To Reduce Fraud Losses and Enhance Customer Trust? Connect With Us! As the banking industry undergoes significant changes through digital transformation services, the adoption of advanced fraud analytics has become critical. These services leverage cutting-edge technology to protect financial institutions from various forms of fraud, ensuring security and trust in an increasingly digital landscape. Here are some key use cases of fraud analytics in banking Credit card fraud detection focuses on identifying unauthorized transactions made with stolen or counterfeit credit card information. Fraud analytics in banking systems analyze transaction patterns to flag unusual activities, such as large purchases or transactions from unfamiliar locations, in real-time. Employ real-time monitoring to detect anomalies such as atypical spending patterns or sudden spikes in transaction volumes. Advanced machine learning models are used to identify subtle signs of fraud that deviate from a cardholder’s typical behavior. Account takeover occurs when fraudsters gain unauthorized access to customer accounts, often through phishing or credential theft. It helps detect and prevent such breaches by monitoring unusual login behaviors and changes in account activity. Utilize behavioral analytics to track login patterns and device usage, flagging any deviations from normal activity. Device fingerprinting and risk-based authentication add additional layers of security by identifying and challenging unfamiliar devices or locations. Insider fraud involves fraudulent activities committed by bank employees or other trusted individuals. Monitoring systems analyze internal transactions and access patterns to detect unauthorized or suspicious activities. Anomaly detection algorithms track unusual transactions or access to sensitive data. Behavioral analysis of employee actions and segregation of duties principles ensure that no single employee has excessive control or access. Loan fraud involves the submission of false information to obtain loans fraudulently. Fraud detection using data analytics in the banking industry aids in preventing this by scrutinizing loan applications for inconsistencies or falsified data. Implement predictive modeling to identify patterns associated with fraudulent applications. Cross-verification with external databases helps validate applicant information, while anomaly detection flags discrepancies in application details. Cyber fraud, including phishing attacks, targets customers through deceptive emails or fake websites. Fraud analytics in banking systems identify these threats by examining email content and web interactions for signs of fraud. Content analysis tools scan communications for known phishing indicators and suspicious content. Behavioral monitoring of user interactions helps detect phishing attempts, while threat intelligence integration provides up-to-date knowledge on emerging cyber threats. AML compliance involves detecting and reporting suspicious activities related to money laundering. Customized fraud analytics solutions analyze financial transactions to identify potential money laundering activities. Transaction monitoring systems analyze transaction volumes and patterns for signs of money laundering, such as unusually large or frequent transactions. Customer profiling helps detect deviations from normal behavior, and suspicious activity reports support regulatory compliance and investigations. Digital channels like online and mobile banking are vulnerable to transaction fraud. Fraud detection using data analytics in the banking industry helps protect these channels by analyzing transaction behaviors and detecting anomalies. Employ device fingerprinting to track and verify devices used for transactions. Velocity checks monitor the speed and frequency of transactions to identify suspicious patterns, while contextual analysis considers factors like transaction location and device type. Also Read: Leveraging Generative AI for Fraud Detection: Improving Accuracy and Reducing False Positives Harness The Power of Fraud Analytics to Upgrade Various Banking Operations! Contact Us! Custom building fraud analytics solutions for banking operations involve several key steps, each critical for ensuring effective implementation. Here’s a detailed look at each step, including how our experts can assist: Also Read: Harnessing the Power of Data Analytics in Banking: A Strategic Investment for Your Business Develop Customized Fraud Analytics Solution to Upgrade Your Business in the Banking Sector! The landscape of fraud analytics in banking is evolving rapidly, driven by advancements in technology and changing fraud tactics. Here are some key future trends: Machine learning and artificial intelligence (AI) are becoming more sophisticated in fraud detection. AI integration services enable deep learning models to analyze complex patterns and detect sophisticated fraud schemes that traditional methods might miss. These adaptive algorithms continuously learn from new data, improving their accuracy over time and reducing the likelihood of false positives. This leads to more precise fraud detection and fewer legitimate transactions being flagged incorrectly. Behavioral analytics is advancing with the development of detailed behavioral profiles for customers based on their typical transaction patterns. Deviations from these profiles can indicate potential fraud. Additionally, incorporating contextual factors such as the device used or the location of transactions enhances the understanding of transactions, allowing for more effective real-time detection and personalized risk assessment. Big data analytics is transforming fraud detection by aggregating extensive data from various sources, including transaction records, social media, and external databases. This comprehensive view helps in refining predictive models, enabling banks to anticipate and mitigate fraud before it occurs. The integration of big data analytics provides a more holistic understanding of customer behavior and improves the ability to take proactive measures against fraud. Cloud-based solutions offer scalability and flexibility, making them increasingly popular for fraud analytics. These platforms can handle large volumes of data and computational needs more efficiently than traditional on-premises solutions. Cloud integration services facilitate easier integration with existing systems and enhance collaboration through centralized access to fraud analytics tools while reducing infrastructure costs. As fraud analytics tools become more advanced, ensuring data privacy and security is critical. Advanced encryption technologies are being implemented to protect sensitive information used in fraud detection. Additionally, compliance with evolving data privacy regulations is becoming more stringent, ensuring that customer data is safeguarded, and regulatory requirements are met. These measures strengthen data security and enhance trust. There is a growing trend towards increased collaboration among banks and financial institutions to share information on fraud trends and threats. Participation in threat intelligence networks provides insights into emerging fraud tactics and techniques, enhancing the collective defense against fraud. This collaborative approach improves awareness of new threats and strengthens the overall fraud detection capabilities of the industry. Fraud analytics in banking is essential for detecting, preventing, and managing fraudulent activities, as well as ensuring the security of financial transactions and customer data. Implementing advanced fraud analytics solutions can significantly enhance your bank’s ability to combat fraud and protect its assets. Here are some key areas where choosing us can be beneficial: You can fill out the form and reach out for our expert’s guidance to explore how you can upgrade your banking operations with fraud analytics solutions. Yes, fraud analytics systems can be seamlessly integrated with both legacy and modern banking infrastructure. We design hybrid solutions that work with your current IT setup to ensure a smooth transition and continuity in your operations. Costs for implementing fraud analytics vary based on factors such as system complexity, technology requirements, and integration needs. We provide clear, detailed estimates tailored to your specific requirements and budget. We offer comprehensive support, including 24/7 assistance, regular system updates, performance optimization, and proactive monitoring. Our goal is to ensure that your fraud analytics system remains effective and up to date. Data migration is managed with a secure and structured approach, involving detailed planning and testing. We use backup and recovery solutions to ensure data integrity and minimize disruption during the transition. We implement robust security measures, including encryption, access controls, and regular audits, to ensure data protection and regulatory compliance. Our approach safeguards sensitive information and maintains the integrity of your fraud analytics system. The Evolution of Banking Fraud
Evolution of Fraudulent Tactics in the Digital Age
Core Components and Methodologies
1. Data Collection and Integration
Data Collection
Data Integration
2. Data Preprocessing
Data Cleaning
Data Transformation
3. Pattern Recognition
Algorithm Development
Anomaly Detection
4. Predictive Modeling
Model Development
Model Validation
Model Deployment
5. Real-Time Monitoring
Response Mechanisms
Feedback Loops
Benefits of Leveraging Fraud Analytics in Banking
Enhanced Fraud Detection
Proactive Prevention
Regulatory Compliance
Customer Trust
Operational Efficiency
Cost Savings
Data-Driven Decision Making
Fraud Analytics Use Cases in Banking
Credit Card Fraud Detection
Account Takeover Prevention
Insider Fraud Monitoring
Loan Fraud Detection
Cyber Fraud and Phishing Detection
AML (Anti-Money Laundering) Compliance
Transaction Fraud Detection in Digital Channels
Steps for Integrating Fraud Analytics Solutions
1. Assess Current Systems
2. Select Appropriate Tools
3. Train Staff
4. Implement Solutions
5. Monitor and Adjust
Future Trends for Fraud Analytics in Banking
Enhanced Machine Learning and Artificial Intelligence
Advanced Behavioral Analytics
Integration of Big Data Analytics
Cloud-Based Solutions
Enhanced Data Privacy and Security Measures
Collaboration and Information Sharing
How Can Matellio Help with Fraud Analytics in Banking?
Q1. Can fraud analytics integrate with existing systems?
Q2. What are the costs associated with implementing fraud analytics?
Q3. What support and maintenance do you offer for fraud analytics?
Q4. How is data migration handled during the implementation of fraud analytics?
Q5. How does Matellio ensure data security and compliance in fraud analytics solutions?