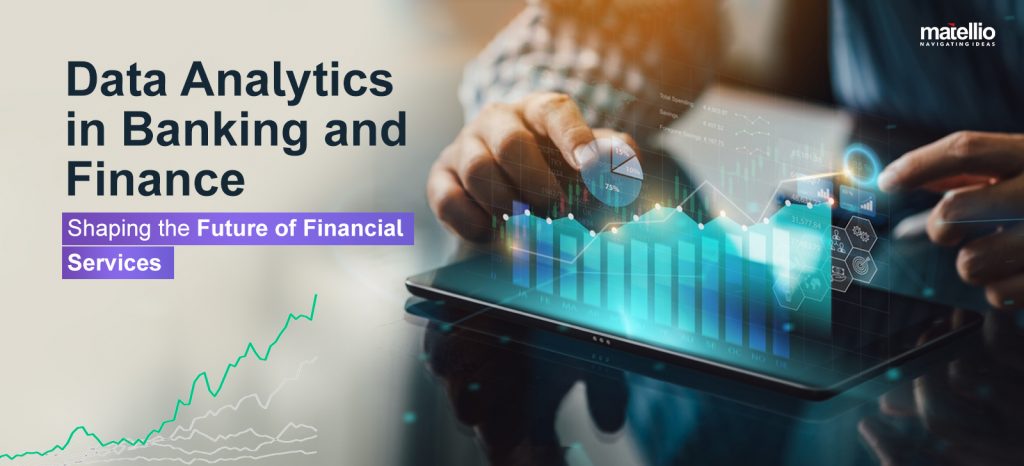
The financial services landscape is undergoing transformation, driven by the rapid adoption of digital technologies. In banking and finance, data analytics is leveraged to enhance customer experiences, streamline operations, and mitigate risks. The integration of advanced analytics tools enables financial institutions to make data-driven decisions, fostering growth and innovation.
This blog delves into the critical aspects of data analytics in banking and finance and how it is reshaping the industry.
Benefits of Data Analytics in Banking and Finance
Enhanced Customer Experience
- Personalized Services: Tailored product recommendations and customized financial advice based on individual customer data.
- Proactive Engagement: Predictive analytics to anticipate customer needs and offer timely solutions, enhancing satisfaction.
- Improved Customer Support: Data-driven insights into customer behavior and preferences enable more responsive and effective customer service through data analytics in banking and finance.
Operational Efficiency
- Process Automation: Automation of routine tasks reduces manual effort, minimizes errors, and speeds up operations.
- Resource Optimization: Data analytics helps efficiently allocate resources, optimize staff schedules, and manage branch operations.
- Cost Reduction: Identifying inefficiencies and streamlining processes leads to significant cost savings, an integral part of digital transformation services.
Risk Management
- Fraud Detection: Advanced analytics tools detect unusual patterns and anomalies in transactions, identifying potential fraud in real-time.
- Credit Risk Assessment: Predictive models assess creditworthiness more accurately, reducing the risk of bad loans.
Competitive Advantage
- Informed Decision-Making: Banking data analytics enables strategic planning and more informed business decisions.
- Market Insights: Understanding market trends and customer preferences helps banks stay ahead of the competition.
Marketing Effectiveness
- Targeted Campaigns: Creating highly targeted marketing campaigns based on detailed customer segmentation.
- ROI Optimization: Analyzing campaign performance to optimize future marketing efforts and improve return on investment.
- Customer Acquisition: Identifying the most effective channels and strategies for attracting new customers using banking data analytics.
Improved Customer Retention
- Enhanced Loyalty Programs: Creating and refining loyalty programs based on customer behavior and preferences.
- Personalized Communication: Tailoring communication and offers to individual customers to enhance engagement and loyalty.
Enhanced Security
- Fraud Prevention: Continuous monitoring and analysis of transactions to prevent fraudulent activities.
- Risk Mitigation: Implementing proactive measures to mitigate various types of risks identified through data analysis.
Financial Planning and Forecasting
- Accurate Forecasting: Leveraging historical data and market trends to improve financial forecasting accuracy.
- Investment Decisions: Making informed investment decisions based on comprehensive data analysis.
- Budgeting: Efficiently allocate resources and set realistic budgets based on data-driven insights facilitated by data analytics in banking and finance.
Better Customer Insights
- Behavior Analysis: Gaining deep insights into customer behavior, preferences, and spending patterns.
- Personalization: Enhancing the ability to offer personalized services and products based on detailed customer profiles.
- Cross-Selling and Upselling: Identifying opportunities for cross-selling and upselling, increasing revenue.
Innovation and Development
- Technology Integration: Integrating advanced analytics tools with existing systems to enhance overall functionality and performance.
- Continuous Improvement: Leveraging data to continuously improve processes, products, and services is a key aspect of data analytics in banking and finance.
Also Read- Transforming Financial Services with Big Data Analytics in Banking
Harness the Power of Data Analytics to Upgrade Your Banking and Finance Operations!
Leveraging Data Analytics in Banking and Finance with Accu-Image
Problem
Accu-Image, a financial tool for managing invoices, encountered inefficiencies and errors due to manual processes. Businesses needed better visibility into payables and streamlined cash flow management.
Solution
Matellio developed a comprehensive digital invoice management system for Accu-Image, incorporating:
- Automated Invoice Processing: Automates creation, sending, and approval of invoices, reducing manual workload and errors.
- Real-Time Data Integration: Uses Plaid API for real-time financial data, enabling informed decision-making.
- Multi-Account Management: Manages multiple business accounts within a single platform.
- Enhanced Security: Ensures secure handling of financial data with robust encryption.
- User-Friendly Mobile Apps: Enables invoice management on the go, with capabilities for uploading, approving, and emailing invoices from mobile devices.
Outcome
The implementation resulted in significant benefits for Accu-Image users:
- Increased Efficiency: Automated processes save time and reduce errors.
- Improved Decision-Making: Real-time data access provides strategic insights.
- Cost-Effective Solutions: Offers a more affordable option compared to traditional bookkeeping methods.
- Enhanced User Experience: Mobile apps provide convenience and flexibility for users to manage invoices anytime, anywhere.
Note: The images above showcase the basic representation of the dashboard and can be changed as per the requirements.
Matellio’s Role
Matellio’s expertise in data analytics enabled the creation of a robust solution that transformed manual invoice management into an efficient, automated process, showcasing their capability in leveraging advanced technologies for banking and finance solutions.
Key Applications of Data Analytics in Banking and Finance
Customer Segmentation and Personalization
Customer Insights
Analyzing customer behavior to create detailed profiles. This involves examining transaction histories, spending patterns, and interaction data to understand customer preferences and needs. Data analytics in banking and finance enables banks to develop a 360-degree view of each customer, facilitating more accurate segmentation.
Product Recommendations
Offering tailored products and services based on individual preferences. By leveraging banking data analytics, banks can recommend relevant financial products, such as loans, credit cards, or investment opportunities, enhancing the customer experience and increasing sales.
Fraud Detection and Prevention
Real-Time Monitoring
Continuous analysis of transactions to detect anomalies. Data analytics in banking and finance allows for the real-time monitoring of all transactions, identifying unusual patterns that could indicate fraudulent activities.
Risk Scoring
Assigning risk scores to transactions for proactive intervention. Each transaction can be evaluated and given a risk score, helping banks to focus their resources on the most suspicious activities.
Credit Risk Assessment
Data-Driven Credit Scoring
Utilizing alternative data sources for accurate credit risk evaluation. Traditional credit scoring methods can be enhanced with additional data sources, such as payment history, to provide a more comprehensive risk assessment.
Predictive Models
Forecasting borrower behavior and potential defaults. Predictive analytics can assess the likelihood of a borrower defaulting on a loan, allowing banks to make more informed lending decisions.
Loan Portfolio Management
Optimizing loan portfolios based on risk analytics. By analyzing the risk associated with each loan, banks can balance their portfolios to minimize risk and maximize returns.
Operational Efficiency
Process Automation
Automating routine tasks to improve efficiency. Banking data analytics can identify tasks that are ripe for automation, reducing manual effort and freeing up staff for more strategic activities.
Resource Optimization
Allocating resources based on predictive demand analysis. Predictive models can forecast customer demand for various services, helping banks to allocate resources more effectively.
Cost Reduction
Identifying cost-saving opportunities through data insights. Data analytics can reveal inefficiencies and areas where costs can be cut without compromising service quality.
Customer Retention and Loyalty
Churn Analysis
Identifying factors leading to customer attrition. By analyzing customer behavior and feedback, banks can pinpoint the reasons why customers leave and take steps to address these issues.
Loyalty Programs
Designing effective loyalty programs based on customer data. Personalized loyalty programs that reward customers based on their preferences and behaviors can significantly enhance customer satisfaction and retention. By leveraging data consultation services, banks can develop and refine these programs to maximize customer engagement and loyalty.
Feedback Analysis
Analyzing customer feedback to improve services. Customer feedback, collected through surveys and social media, can be analyzed to identify areas for improvement and enhance customer satisfaction.
Regulatory Compliance
Compliance Monitoring
Ensuring adherence to regulatory requirements through continuous monitoring. Data analytics can automate the monitoring of transactions to ensure they comply with regulations, reducing the risk of non-compliance.
AML Analytics
Detecting and preventing money laundering activities. Anti-money laundering (AML) analytics tools can identify suspicious transactions that may indicate money laundering, helping banks stay compliant with regulations.
Regulatory Reporting
Automating the generation of compliance reports. Data analytics in banking and finance can streamline the reporting process by automatically generating reports required by regulatory authorities, ensuring accuracy and timeliness.
Want to Hire Experts for Custom-Building Data Analytics Solution for Banking and Finance?
Challenges in Implementing Data Analytics in Banking and Finance
Data Quality and Integration
- Challenge: Ensuring the quality and consistency of data across various sources can be difficult. Inconsistent data can lead to inaccurate analytics and poor decision-making.
- Solution: Implementing robust data cleansing and integration processes is essential. Custom-built data analytics solutions address this by automating data validation and harmonizing data from diverse sources, ensuring accuracy and consistency. Cloud integration services can facilitate seamless data flow across platforms, enhancing data quality management.
Data Security and Privacy
- Challenge: Protecting sensitive customer information and ensuring compliance with data privacy regulations is crucial in banking and finance.
- Solution: Advanced encryption and access control measures are integrated into custom data analytics solutions to enhance data security and privacy. Solutions designed with the latest security protocols help banks safeguard customer data and meet regulatory compliance requirements.
Read More: Discover how Customer Data Analytics Tools empower businesses with actionable insights and drive data-driven decisions for enhanced growth.
Integration with Legacy Systems
- Challenge: Integrating modern analytics tools with existing legacy systems can be complex and disruptive.
- Solution: Custom integration solutions bridge the gap between new analytics technologies and legacy systems, ensuring smooth data flow and interoperability. Effective data analytics in banking and finance solutions are designed to integrate with legacy systems without significant disruption.
Scalability
- Challenge: As the volume of data grows, scaling data analytics solutions to handle increased loads can be challenging.
- Solution: Custom-built solutions offer scalable architectures that accommodate growing data volumes and evolving analytics needs. Implementing cloud-based platforms and scalable databases ensures that the analytics infrastructure can grow with the bank’s needs.
Change Management
- Challenge: Implementing custom solutions for data analytics in banking industry requires significant changes in workflows and processes, which can face resistance from employees.
- Solution: Effective change management strategies, including training and stakeholder engagement, ensure a smooth transition to new analytics systems.
Data Governance
- Challenge: Establishing robust data governance frameworks to ensure data quality, security, and compliance is essential.
- Solution: Custom-built solutions often incorporate data governance tools and practices to ensure that data is managed effectively and in compliance with regulations. This includes automated compliance checks and data stewardship programs.
Talent Acquisition
- Challenge: Finding skilled data scientists and analysts who understand both analytics and banking can be difficult.
- Solution: Custom-built solutions often come with expert support and training to help banks build in-house analytics capabilities. Partnering with analytics service providers can also provide access to specialized talent and knowledge transfer.
Struggling with Legacy Systems? Develop Modern Software & Gain Efficiency.
Future Trends in Data Analytics in Banking and Finance
Advanced Analytics and Machine Learning
Predictive Analytics
Leveraging machine learning to forecast future trends and customer behaviors. Advanced analytics tools can analyze historical data to predict future events, helping banks to make proactive decisions.
AI-Driven Insights
Utilizing artificial intelligence to gain deeper insights and automate complex decision-making processes. AI can uncover hidden patterns in data that traditional analytics methods might miss, providing banks with valuable insights facilitated by AI integration services.
Real-Time Analytics
Enabling real-time data analysis for faster decision-making. Real-time analytics tools can process and analyze data as it is generated, allowing banks to respond to events immediately.
Enhanced Customer Insights
360-Degree Customer View
Integrating data from various sources to create a comprehensive view of each customer. Enhanced customer insights enable banks to understand their customers better and deliver personalized services.
Behavioral Analytics
Analyzing customer behavior to anticipate needs and offer proactive solutions. By understanding customer behavior, banks can predict future actions and tailor their services accordingly.
Cybersecurity Analytics
Threat Detection
Utilizing advanced analytics to detect and prevent cyber threats. Cybersecurity analytics tools can identify suspicious activities and potential threats, ensuring the security of banking systems.
Anomaly Detection
Identifying unusual patterns that could indicate security breaches. Anomaly detection algorithms can spot deviations from normal behavior, helping to prevent cyber-attacks.
Incident Response
Enhancing incident response capabilities through real-time data analysis. Real-time analytics enable banks to respond quickly to security incidents, minimizing damage.
Open Banking
API Integration
Facilitating seamless data sharing through APIs. Open banking enables banks to share data with third-party providers through secure APIs, fostering innovation and collaboration, particularly when combined with AI in Banking.
Third-Party Services
Integrating third-party services to offer customers a wider range of financial products. Open banking allows banks to partner with fintech companies to provide innovative services.
Customer Empowerment
Giving customers control over their financial data. Open banking empowers customers to choose the services that best meet their needs.
Personalization and Customer Experience
Hyper-Personalization
Leveraging data analytics to provide highly personalized financial products and services. By analyzing customer data, banks can tailor their offerings to individual needs and preferences, improving customer satisfaction and loyalty.
Customer Journey Analytics
Mapping out and analyzing the entire customer journey to identify pain points and opportunities for improvement. This helps banks enhance the overall customer experience and optimize service delivery.
Financial Inclusion
Credit Scoring with Alternative Data:
Using data analytics in banking and finance to develop credit scores based on non-traditional data sources, such as social media and utility payments. This helps banks extend credit to underserved populations and promote financial inclusion.
Microfinance Analytics
Leveraging data analytics to optimize microfinance services. By analyzing data on microloan recipients, banks can improve the effectiveness of microfinance programs and support economic development.
Green Finance and Sustainability
Sustainability Analytics
Using data analytics to assess and improve the environmental impact of financial activities. Banks can analyze their portfolios to identify opportunities for sustainable investments and reduce their carbon footprint.
Green Finance Initiatives
Leveraging data analytics to support green finance initiatives. By analyzing data on environmental impact, banks can develop and promote financial products that support sustainability goals.
How Can Our Experts Help Implement Data Analytics in Banking and Finance?
Data analytics in banking and finance is transforming the industry by providing deeper insights, enhancing customer experiences, and improving operational efficiencies. Our experts can assist financial institutions in several critical areas to ensure successful implementation and maximize the potential of data analytics:
- Our approach involves closely collaborating with each client to develop customized data analytics solutions that align with their specific objectives and requirements.
- Utilizing technologies such as the Plaid API, we enable financial institutions to access up-to-date financial information in real-time. This capability is crucial for making timely and informed decisions, improving responsiveness to market changes, and enhancing overall financial management.
- Our technology consulting services play a significant role in helping financial institutions implement data analytics effectively. We provide expert advice on technology selection, system integration, and process optimization to ensure that the chosen solutions align with the institution’s strategic goals and technical environment.
Feel free to contact us today by filling out the form to learn more about how we can empower your business with data analytics.
FAQs
Q1. Can data analytics in banking and finance integrate with existing systems?
Yes, we specialize in creating hybrid solutions that seamlessly integrate data analytics with both legacy systems and modern architectures. This approach allows you to leverage the benefits of data analytics while preserving your current IT investments.
Q2. What are the costs associated with data analytics in banking and finance services?
The costs for data analytics services in banking and finance vary based on factors such as project complexity, technology requirements, customization needs, and integration specifications. We provide transparent cost estimates tailored to your specific organizational needs, ensuring a solution that aligns with your budget and strategic objectives.
Q3. What support and maintenance do you offer for data analytics in banking and finance?
Our team provides comprehensive support throughout the integration process, including 24/7 technical assistance, regular updates, performance optimization, and proactive monitoring. Our goal is to ensure that your data analytics system operates smoothly, remains up to date with technological advancements, and delivers reliable and actionable insights.
Q4. What technologies are typically used in data analytics for banking and finance?
Technologies used in data analytics for banking and finance include data lakes, Hadoop, Spark, machine learning platforms, and advanced visualization tools. These technologies collectively enhance the processing, analysis, and visualization of large datasets, enabling more accurate and timely insights.
Q5. How long does it take to implement a data analytics solution in a financial institution?
The implementation timeline for a data analytics solution can vary based on the project's scope, complexity, and specific requirements. Typically, the process involves several phases, including planning, infrastructure setup, data integration, tool deployment, and staff training, and can take several months to complete.