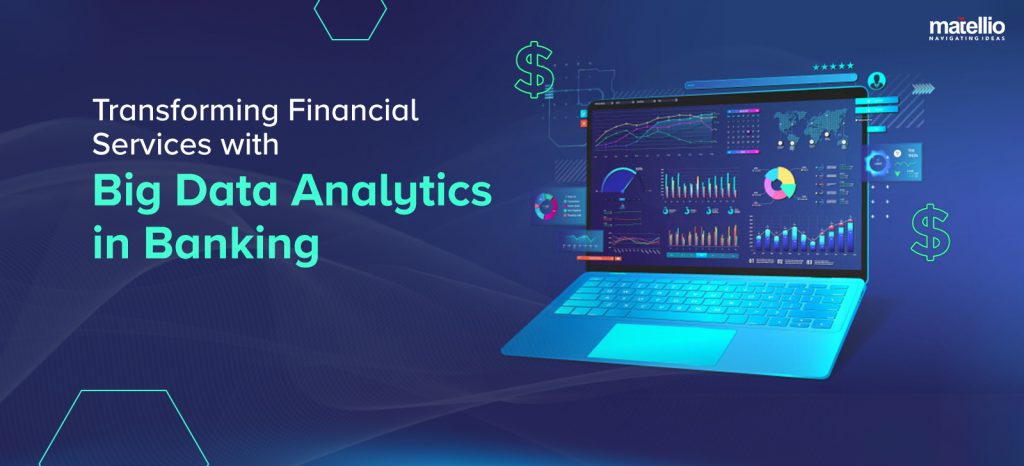
In the rapidly evolving financial sector, big data analytics in banking has emerged as a transformative force. By harnessing vast amounts of data, banks can gain unprecedented insights into customer behavior, enhance risk management, streamline operations, and innovate new products and services. This blog explores how big data analytics is reshaping the banking industry and driving it toward a more efficient and customer-centric future.
Understanding Big Data Analytics in Banking
Big data analytics in banking industry refers to the process of examining large and varied data sets. Unlike traditional data analytics, big data analytics handles massive volumes of data generated at high velocity from various sources, including transactional data, social media, and machine-generated data.
Components of big data analytics in banking include:
Volume
Managing large quantities of data. Banks generate vast amounts of data daily from transactions, customer interactions, social media, and other sources. Efficiently storing, processing, and analyzing this massive volume is crucial. To handle this effectively, banks often leverage data consultation services to design and implement scalable data storage and processing solutions.
Velocity
Processing data at high speed. Financial transactions and market movements happen in real time. Big data analytics enables banks to process and analyze data rapidly, allowing them to make timely decisions and respond to market changes instantly. Fast data processing is essential for activities like fraud detection, where immediate action is required.
Variety
Handling different types of data, both structured and unstructured. Data in banking comes in various forms, including structured data (like transaction records and customer details) and unstructured data (such as social media posts and customer feedback). Big data analytics tools can handle and integrate these diverse data types, providing a comprehensive view that supports better decision-making.
Wish to Learn More About the Components of Big Data Analytics?
Contact Us Now!
How Big Data Analytics is Changing the Banking Sector?
Customer Insights
Big data analytics in banking enables institutions to gain a deeper understanding of customer needs and behaviors. By analyzing data from various sources, such as transactional history, social media interactions, and customer feedback, banks can personalize their services, enhance customer satisfaction, and build stronger relationships.
- Segmentation and Targeting: Big data allow banks to segment their customer base more accurately, enabling more targeted marketing efforts and personalized communications.
- Customer Journey Mapping: By tracking and analyzing customer interactions across various touchpoints, banks can improve the overall customer experience and identify areas for enhancement.
Enhanced Risk Management
Leveraging big data, banks can better predict and manage risks. This includes identifying potential defaults, assessing creditworthiness, and detecting fraudulent activities in real time. By integrating big data analytics with AI in banking, institutions can enhance their risk management capabilities even further. AI algorithms can analyze vast datasets at high speed, identifying subtle patterns and anomalies that might indicate risk.
- Credit Risk Modeling: Big data analytics helps in building more accurate credit risk models by incorporating a wider range of data points, such as payment histories and social media activity.
- Fraud Detection: Advanced analytics and AI can detect unusual transaction patterns and flag potentially fraudulent activities in real time, enabling faster response and mitigation.
Operational Efficiency
Big data analytics in banking sector helps banks improve internal processes and reduce costs. By optimizing workflows and automating routine tasks, banks can increase efficiency and productivity. This includes everything from streamlining loan approval processes to improving customer service operations.
- Process Automation: Automating repetitive tasks with big data analytics and AI reduces manual errors and frees employees to focus on more strategic activities.
- Resource Optimization: Data analytics helps in efficient resource allocation by predicting demand and aligning workforce management accordingly.
Read More: How AaaS (Analytics as a Service) is revolutionizing data-driven decision making for modern businesses, explore our blog on Analytics as a Service
Product Innovation
Banks can develop new products and services based on insights gained from big data analytics. This leads to more relevant offerings that meet the evolving needs of customers. By understanding customer preferences and market trends, banks can create innovative financial products that cater to specific segments.
- Custom Financial Products: Data-driven insights enable banks to design customized products, such as personalized loan packages or investment plans.
- Market Analysis: Big data analytics provides a detailed understanding of market conditions, helping banks to innovate and adapt quickly to changing market demands.
Regulatory Compliance
Big data analytics helps banks ensure compliance with regulatory requirements by providing detailed and accurate reporting capabilities.
- Automated Reporting: Big data tools can automate the generation of compliance reports, ensuring timely and accurate submissions.
- Regulatory Risk Management: Analytics helps in monitoring compliance-related risks and implementing proactive measures to address them.
Improved Decision Making
Data-driven decision-making is one of the most vital advantages of big data analytics banking. By providing actionable insights, big data helps bank executives make informed strategic decisions.
- Strategic Planning: Comprehensive data analysis aids in strategic planning and forecasting, ensuring that banks are better prepared for future challenges.
- Real-time decision-making: Real-time analytics provide immediate insights, allowing banks to respond swiftly to emerging trends and issues.
Customer Retention
Predictive analytics can identify customers who are likely to leave, allowing banks to take proactive measures to retain them.
- Churn Analysis: By understanding the reasons behind customer churn, banks can implement targeted retention strategies.
- Loyalty Programs: Data analytics helps in designing effective loyalty programs that resonate with customers and encourage long-term relationships.
Harness The Power of Big Data Analytics to Sustain Your Business Growth!
Connect With Us
Use Cases of Big Data Analytics in Banking
Real-time Fraud Detection
By analyzing transactions in real time, banks can detect and prevent fraud, protecting their assets and customer trust. Advanced algorithms and machine learning models can identify unusual patterns and flag potentially fraudulent activities, enabling immediate action.
Credit Scoring
Big data analytics in banking enables the development of more accurate credit scoring models by incorporating a wider range of data points, such as social media activity, purchasing behaviors, and other non-traditional credit indicators. This leads to a more comprehensive assessment of creditworthiness.
Customer Churn Prediction
Identifying patterns that indicate potential customer churn helps banks take proactive measures to retain customers. By analyzing customer behavior, transaction history, and engagement levels, banks can implement targeted retention strategies and improve customer loyalty.
Market Trend Analysis
Analyzing market data allows banks to identify trends and opportunities, enabling them to stay ahead of the competition. This includes monitoring economic indicators, industry news, and consumer behavior to inform strategic decisions.
Predictive Maintenance
Banks can anticipate equipment failures and schedule maintenance proactively, minimizing downtime and operational disruptions. This is particularly useful for maintaining ATMs, servers, and other critical infrastructure.
Personalized Marketing Campaigns
Using big data analytics in banking industry can create highly personalized marketing campaigns tailored according to customer preferences and behaviors. It increases the effectiveness of marketing efforts and improves customer engagement.
Loan Default Prediction
By analyzing customer data, transaction history, and economic conditions, banks can predict the likelihood of loan defaults. This allows banks to manage their loan portfolios more effectively and take preventive measures to mitigate risks.
Customer Lifetime Value (CLV) Analysis
Big data analytics helps banks calculate the expected value generated from a customer over their entire relationship with the bank. This information is used to prioritize resources and marketing efforts on high-value customers.
Risk Management and Mitigation
Big data analytics allows banks to assess and manage a variety of risks, including market risk, credit risk, and operational risk. Historical data and current market conditions are analyzed and then the strategies can be developed to mitigate potential risks and protect their assets.
Operational Efficiency
By leveraging big data analytics, banks can streamline internal processes and reduce operational costs. For instance, data-driven insights can optimize workforce management, improve process workflows, and enhance overall productivity. Integrating big data analytics as part of digital transformation services ensures that banks modernize their operations for greater efficiency and competitiveness.
How to Implement Big Data Analytics in Banking Sector?
Data Strategy
Developing a data strategy is significant for the successful implementation of big data analytics in banks. The strategy is aligned with the bank’s overall business goals.
- Define Objectives: Clearly outline what the bank aims to achieve with big data analytics, such as improving customer experience, enhancing risk management, or increasing operational efficiency.
- Data Governance: Establish policies and procedures to ensure data quality, security, and compliance.
- Technology Roadmap: Develop a roadmap for implementing big data technologies and integrating them with existing systems, ensuring a cohesive approach to data analytics.
Also Read: Harnessing the Power of Data Analytics in Banking: A Strategic Investment for Your Business
Infrastructure Setup
Establishing the necessary IT infrastructure is essential to support big data analytics. This includes ensuring adequate storage, processing power, and network capabilities.
- Scalable Storage Solutions: Implement scalable storage solutions, such as data lakes, to handle the vast volumes of data generated by banks.
- Processing Power: Invest in high-performance computing resources to process and analyze large datasets efficiently.
- Network Capabilities: Ensure robust network infrastructure to support the seamless transfer and accessibility of data.
- Integration Services: Utilize cloud integration services to enhance flexibility, scalability, and cost-effectiveness. Cloud platforms can provide the necessary infrastructure to handle big data workloads efficiently.
Data Integration
Integrating data from various sources into a unified platform is critical for comprehensive analysis. This step ensures seamless data flow and accessibility across the organization.
- Data Sources: Identify and catalog all relevant data sources, including transactional data, customer interactions, social media, and third-party data.
- ETL Processes: Implement Extract, Transform, and Load (ETL) processes to consolidate and standardize data from different sources.
- Unified Data Platform: Develop a unified data platform that supports real-time data integration and analytics. This platform should enable easy access to data for various analytical purposes.
Advanced Analytics Tools
Selecting and deploying advanced big data analytics tools that align with the bank’s specific needs and objectives is crucial.
- Tool Evaluation: Assess different big data analytics tools based on their capabilities, scalability, and compatibility with the bank’s existing IT infrastructure.
- AI Integration Services: Integrate AI capabilities into your analytics tools to enhance predictive analytics, automate decision-making, and uncover deeper insights from data. Leveraging AI integration services can significantly boost the analytical power of big data tools.
- User-Friendly Interfaces: Choose tools that offer intuitive interfaces and visualization capabilities to make data analysis accessible to non-technical users.
Talent Acquisition
Hiring or training staff with expertise in big data analytics is essential to ensure the effective use of analytics tools and techniques. Our experts provide guidance to build a skilled team capable of maximizing the impact of big data analytics.
- Skill Assessment: Identify the specific skills and expertise required for big data analytics within the bank. Our team can help assess current capabilities and identify skill gaps that need to be addressed.
- Recruitment: Hire data scientists, data engineers, and analysts with the necessary skills and experience. With our assistance, you can attract top-tier talent with the right qualifications.
- Training Programs: Develop training programs to upskill existing employees on big data analytics tools and methodologies. Partnering with our experts ensures access to customized training programs tailored to your bank’s specific needs.
Continuous Improvement
Continuously refining and optimizing big data analytics processes is crucial to adapt to new data and evolving market conditions.
- Performance Monitoring: Regularly monitor the performance of big data analytics initiatives using key performance indicators (KPIs) to identify areas for improvement.
- Feedback Loops: Establish feedback loops to incorporate insights from analytics into business strategies and operations.
- Innovation: Stay abreast of the latest developments in big data analytics technologies and methodologies to ensure the bank remains competitive and innovative.
Get Experts Guidance on How to Implement Big Data Analytics in the Banking Industry!
Fill out The Form
How Can Matellio Help Implement Big Data Analytics in Banking?
Big data analytics in banking is revolutionizing the industry by providing deeper insights, enhancing customer experiences, and improving operational efficiencies. As banks continue to adopt and integrate big data analytics, they can expect to see significant benefits. Our experts can assist banks in several critical areas to ensure successful implementation and maximize the potential of big data analytics.
- Our team of experts has extensive experience in implementing big data analytics solutions in the banking industry.
- We don’t believe in one-size-fits-all solutions. Our approach is to work closely with each bank to understand its unique goals and challenges, providing customized solutions that align with its specific objectives and requirements.
- From strategic planning to implementation and continuous improvement, we offer end-to-end support to ensure the successful deployment of big data analytics.
- The technology consulting services that we offer helps banks to implement big data analytics effectively. We provide expert advice on technology selection, system integration, and process optimization to ensure that the chosen solutions align with the bank’s strategic goals and technical environment.
Feel free to contact us today by filling out the form to learn more about how we can empower your business with data analytics.
FAQs
Q1. Can big data analytics in banking integrate with existing systems?
Yes, we specialize in creating hybrid solutions that seamlessly integrate big data analytics with both legacy systems and modern architectures. This approach allows you to leverage the benefits of big data analytics in banking while preserving your current IT investments.
Q2. What are the costs associated with big data analytics in banking services?
The costs for big data analytics in banking services vary based on factors such as project complexity, technology requirements, customization needs, and integration specifications. We provide transparent cost estimates tailored to your specific organizational needs, ensuring a solution that aligns with your budget and strategic objectives.
Q3. What support and maintenance do you offer for big data analytics in banking?
Our team provides comprehensive support throughout the integration process, including 24/7 technical assistance, regular updates, performance optimization, and proactive monitoring. Our goal is to ensure that your big data analytics system in banking operates smoothly, remains up to date with technological advancements, and delivers reliable and actionable insights.
Q4. What technologies are typically used in big data analytics for banking?
Technologies used in big data analytics for banking include data lakes, Hadoop, Spark, machine learning platforms, and advanced visualization tools. Cloud integration services and AI integration services are also commonly utilized to enhance the scalability and analytical capabilities of big data solutions.
Q5. How long does it take to implement a big data analytics solution in a bank?
The implementation timeline for a big data analytics solution can vary based on the project's scope, complexity, and specific requirements. Typically, the process involves several phases, including planning, infrastructure setup, data integration, tool deployment, and staff training, and can take several months to complete.