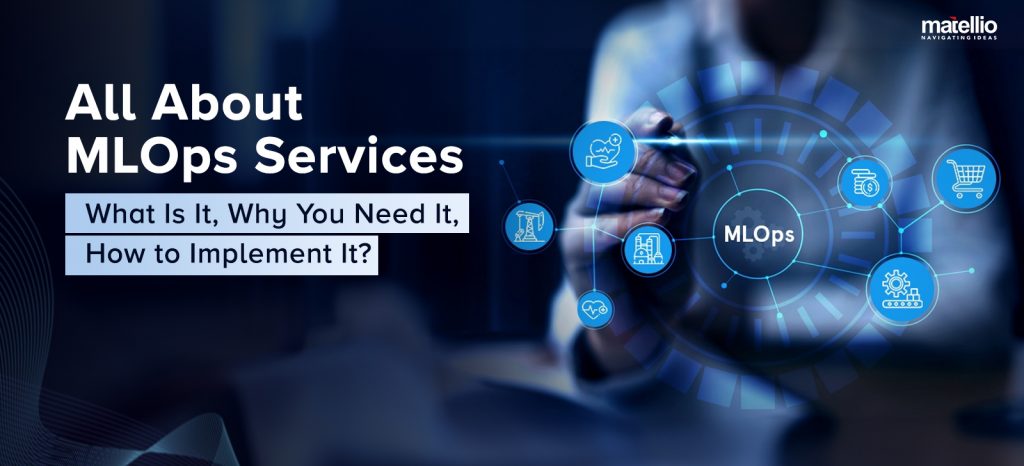
What is MLOps and why do we need it?
Well, if you are searching for the answer to this question, you are at the right place!
In today’s lightning-fast digital landscape, machine learning isn’t just an advantage—it’s the lifeline of modern business. But here’s the stark reality: up to 87% of machine learning projects never make it to production. Why? The answer lies in a critical gap that’s costing companies millions: MLOps services.
MLOps services (Machine Learning Operations) is the bridge between groundbreaking AI innovations and real-world business impact. It’s not just another tech buzzword; it’s the make-or-break factor determining whether your AI investments soar or plummet.
Imagine this: Your competitors are deploying AI models in days, not months. They’re slashing operational costs by 30% while you’re still wrestling with model drift. They’re making data-driven decisions at the speed of thought, while you’re stuck in endless deployment cycles. This isn’t a dystopian future—it’s happening right now to businesses without robust MLOps software.
But here’s the game-changer: With the right MLOps services, you can:
- Accelerate time-to-market for AI solutions
- Reduce model errors that directly impact your bottom line
- Achieve seamless scalability, handling millions of predictions without breaking a sweat
- Ensure ironclad compliance and governance in an increasingly regulated AI landscape
The truth is, in the AI-driven economy, MLOps services aren’t optional—it’s existential. Companies without it are not just falling behind; they’re at risk of becoming obsolete.
So, why not adopt MLOps services for your company? In this blog, we will find answers to all the questions like what is MLOps and why do we need it? We will also explore some amazing benefits of MLOps platform and how you can implement it in your company.
What is MLOps?
So, what is MLOps, and how does it apply to your business? MLOps, or Machine Learning Operations, is a set of best practices and processes that bridges the gap between data science and IT operations. It allows companies to seamlessly develop, deploy, monitor, and maintain machine learning models in production environments. Essentially, MLOps services enable businesses to scale their custom machine learning solutions from proof-of-concept to full-scale production, ensuring that models not only work but thrive in real-world scenarios.
Think of MLOps as the glue that holds together every stage of your machine learning lifecycle—from data collection and model training to deployment and performance monitoring. Without MLOps platforms, your machine learning models are at risk of stagnation, suffering from challenges like model drift, inefficient deployment cycles, and security vulnerabilities. MLOps services bring automation, collaboration, and governance to the table, transforming isolated ML experiments into business-driving solutions.
However, MLOps is not a one-time process; it involves several key stages that ensure the successful lifecycle of a machine learning model. Here’s a quick overview of the stages involved:
Model Development
This is where data scientists build, and train models based on business needs. In this stage, MLOps ensures collaboration between data scientists, engineers, and other stakeholders by automating data preprocessing and model versioning.
Model Validation
Once the model is developed, it’s crucial to validate its performance using testing data. MLOps helps automate this stage by running experiments and comparing the performance of different models, ensuring that only the best version moves forward.
Model Deployment
The validated model is deployed into production environments. Here, MLOps automates deployment pipelines, ensuring seamless integration with business systems. Models can be scaled up or down based on user demand.
Monitoring and Retraining
The work doesn’t stop once the model is live. MLOps solutions constantly monitor model performance, identifying when the model starts to drift due to new data patterns. If the performance deteriorates, the model is automatically retrained to ensure it continues delivering value.
Governance and Security
Throughout the entire process, MLOps ensures that every action is tracked, logged, and compliant with regulations. This stage includes safeguarding sensitive data, auditing model decisions, and ensuring full traceability of all changes.
By following these stages, MLOps services allow businesses to transform their machine learning projects into fully operational, scalable, and secure solutions.
Why Do You Need MLOps?
Why do we need MLOps?
Well, this can be a very general question for all business owners who are reading this blog right now! But here’s the answer.
If your business is serious about leveraging machine learning for real-world impact, MLOps consulting services are not just an option—they’re essential.
Also Read- Machine Learning as a Service (MLaaS): Everything a Business Needs to Know
In today’s fast-paced digital landscape, the demand for deploying machine learning models quickly, efficiently, and at scale is higher than ever. Without the right MLOps solution, you’re setting yourself up for failure. Think about it—what good is a cutting-edge AI model if it never makes it out of the testing phase?
Well, that’s what market stats tell about MLOps services and solutions!
In a 2022 survey, 85% of organizations already had a dedicated budget for MLOps, with an additional 14% planning to allocate funds in 2023. This shows how crucial MLOps services are becoming across industries.
Clearly, without MLOps services, you’re likely wasting valuable time and resources, and even worse—losing ground to competitors who are already leveraging these tools. Here’s why MLOps is critical for your business:
Speed and Efficiency
Imagine deploying machine learning models in days, not months. With MLOps platforms, you can drastically reduce the time it takes to move from development to production. MLOps software automates model deployment, so you’re not stuck in endless cycles of manual testing and debugging.
Scalability
Without an MLOps solution, scaling your machine learning models across multiple environments—staging, production, or across geographies—becomes a nightmare. MLOps as a service enables you to scale effortlessly, handling millions of predictions while maintaining model performance.
Reduced Errors and Increased Accuracy
Manual processes are prone to errors, especially in a complex field like machine learning. With MLOps consulting, you automate the monitoring and retraining of models, ensuring they perform optimally and without frequent intervention.
Cost Efficiency
Every delay in model deployment or retraining costs your business. MLOps platforms help you reduce operational costs by automating routine tasks and allowing your team to focus on innovation rather than maintenance. By using MLOps software, you save money by preventing costly mistakes like model drift, data leaks, or compliance violations.
Cross-Team Collaboration
One of the major MLOps challenges is enabling collaboration between data science teams and IT operations. With MLOps services, you create a collaborative environment where data scientists, developers, and operations teams work in sync, leading to faster and smoother deployments.
Compliance and Security
In today’s world of stringent regulations, your machine learning models need to comply with data governance and security standards. MLOps platforms ensure that your models meet all compliance requirements, and they offer secure data management frameworks to keep sensitive information safe.
The Bottom Line: You Can’t Afford to Ignore MLOps
In the AI-driven economy, your competitors are already leveraging MLOps services to deploy models faster, reduce costs, and stay ahead of the curve. Can your business afford to lag behind? The truth is, without an effective MLOps solution, your machine learning investments will fall flat. Delayed deployments, operational inefficiencies, and compliance risks are just a few of the dangers you face by not adopting MLOps.
So why wait? By implementing MLOps consulting services, you can transform your machine learning projects into business-driving solutions that give you a competitive edge. The time to act is now—because without MLOps, your AI investments are at risk of becoming irrelevant.
Let’s Begin Implementing MLOps in Your Business with a Free 30-Minute Consultation!
What is the Use of MLOps?
Why and when to employ MLOps
Well, you have certainly found the answer to why do we need MLOps. But when to employ MLOps. So, here’s the answer!
The use of MLOps services (Machine Learning Operations) extends far beyond simply managing machine learning models—it’s about turning those models into real, measurable business outcomes. MLOps services help you ensure that your machine learning (ML) models operate efficiently and effectively throughout their lifecycle, from development to deployment and ongoing monitoring. Here’s how MLOps services can benefit your business:
Automating Model Deployment
One of the primary uses of MLOps platforms is to automate the deployment process for ML models. Traditionally, getting a model from the data science team to a production environment can be slow and riddled with errors. MLOps solutions automate and streamline this process, reducing manual steps, eliminating inefficiencies, and accelerating time-to-market for AI applications. This is crucial for businesses that need to rapidly implement their models to stay competitive.
Continuous Monitoring and Maintenance
After deploying a model, the next challenge is ensuring it continues to perform as expected. MLOps software is used to constantly monitor model performance, track accuracy, and detect issues like model drift (where models become less effective over time due to changing data patterns). By detecting these problems early, businesses can trigger automated retraining, ensuring models stay effective and reliable.
Improving Collaboration Across Teams
MLOps services enhance collaboration between data scientists, IT, and operations teams. Through automated workflows and centralized tools, everyone involved in the model’s lifecycle—from development to deployment—can easily collaborate, share updates, and manage changes in real time. This ensures that all stakeholders are on the same page, leading to faster and more efficient project execution.
Scaling Machine Learning Models
As businesses grow, the ability to scale ML models across different environments and locations becomes critical. MLOps platforms enable seamless scalability, allowing companies to handle an increasing number of predictions, models, and data sources without losing performance. For example, as your company grows from 100 users to 10,000, MLOps ensures your models continue to perform optimally without disruptions.
Ensuring Compliance and Security
In highly regulated industries, data security and compliance are critical. MLOps solutions ensure that models are built, deployed, and managed in line with data privacy regulations and company policies. This includes secure data handling, audit trails, and automated governance processes, ensuring your machine learning operations are compliant with legal and industry standards.
Optimizing Costs
Running machine learning models can be resource-intensive, leading to high operational costs. MLOps services optimize the entire ML lifecycle by automating repetitive tasks like model retraining and performance tracking. This reduces human error and cuts down on the time and resources required to manage ML models, ultimately leading to lower operational costs.
In summary, the use of MLOps services transforms machine learning initiatives from a series of isolated experiments into fully operational, scalable, and secure business tools. Whether you’re aiming to speed up deployment, ensure long-term model performance, or keep costs in check, MLOps is the key to making your machine learning investments deliver real, tangible results.
What is the Difference Between MLOps and DevOps?
While MLOps services and DevOps consulting services share similarities in their goal of improving operational efficiency, there are key differences between the two. Both focus on streamlining processes, automating workflows, and ensuring collaboration, but they cater to different operational needs. Here’s a breakdown of the major differences:
Difference Between DevOps and MLOps,
Aspect | MLOps | DevOps |
Focus | Machine learning model lifecycle | Software development and deployment |
Data vs. Code | Handles both data and code | Focuses mainly on code |
Complexity | Involves data collection, model training, deployment, retraining | Involves code integration, deployment, infrastructure |
Collaboration | Data scientists, ML engineers, IT operations | Developers and IT operations |
Monitoring | Model performance and retraining | Application performance and infrastructure health |
Core Focus
MLOps (Machine Learning Operations)
Primarily focuses on managing the lifecycle of machine learning models, from development and training to deployment, monitoring, and retraining. MLOps services address challenges unique to ML, like handling vast datasets, retraining models when data patterns change, and ensuring model governance and compliance.
DevOps (Development Operations)
Focuses on improving collaboration between software development (Dev) and IT operations (Ops). It primarily deals with code integration, application deployment, continuous delivery, and infrastructure management. The goal of DevOps is to speed up the software development lifecycle while ensuring high-quality software delivery.
Also Read- DevOps Implementation for Business – A Complete Guide
Data vs. Code
MLOps
Involves both data and code. Managing data is as important as managing the machine learning models themselves. MLOps must handle versioning of both datasets and models, ensuring that the models are retrained and updated with new data over time.
DevOps
Focuses mainly on code. While DevOps handles infrastructure automation, CI/CD pipelines, and application monitoring, it doesn’t deal with the complexities of data management or model performance like MLOps does.
Lifecycle Complexity
MLOps
The lifecycle in MLOps is more complex, involving multiple stages—data collection, model training, model validation, deployment, and continuous retraining based on new data. MLOps requires constant monitoring of model performance to address issues like model drift (where the model’s performance degrades over time due to changing data patterns).
DevOps
The lifecycle in DevOps is focused on streamlining the software development and deployment process, emphasizing CI/CD (Continuous Integration/Continuous Deployment). DevOps is mainly concerned with automating the process of integrating and deploying code into production environments.
Collaboration
MLOps
Fosters collaboration between data scientists, machine learning engineers, and IT operations teams. MLOps solutions require the integration of data pipelines, model training, and model retraining into the operational workflow.
DevOps
Brings together software developers and IT operations teams to collaborate on application development and deployment. The focus is on automating infrastructure and ensuring the smooth deployment of code across environments.
Monitoring and Feedback
MLOps
Monitors model performance in production, checking for accuracy, drift, and degradation. The feedback loop involves constant retraining of models using new data to ensure performance doesn’t degrade.
DevOps
Primarily monitors application performance (e.g., uptime, latency, errors) and infrastructure health. The feedback focuses on fixing bugs and improving application performance rather than retraining models.
In essence, while both MLOps and DevOps aim to improve operational efficiency, MLOps is tailored to the complexities of machine learning, focusing on data, model training, and continuous optimization, while DevOps is more about streamlining software development and deployment.
Confused Between DevOps and MLOps? Let Our Experts Help You Over a Free 30-Minute Consultation Call!
How to Implement MLOps?
Implementing MLOps services can greatly enhance the efficiency and scalability of your machine learning operations, and there are several approaches based on the maturity of your company’s machine learning efforts. According to Google, MLOps can be implemented at three distinct levels—each level increasing in automation and operational efficiency.
MLOps Level 0: Manual Process
At MLOps level 0, machine learning workflows are managed manually.
This is typical for organizations that are just starting with machine learning consulting and have a limited need for frequent retraining of models. While this approach might suffice early on, it introduces major MLOps challenges as models scale or need frequent updates.
Characteristics
- Manual Execution: Each stage of the ML lifecycle, from data preparation to deployment, is managed manually with no MLOps platform in place.
- Disconnected Teams: Data scientists develop models, which are then manually handed over to engineers for deployment, with minimal collaboration.
- No CI/CD: Continuous integration (CI) and continuous deployment (CD) processes are non-existent, and models are rarely updated.
- No Automated Monitoring: Without automated tools, model performance isn’t tracked, which can lead to outdated predictions.
Challenges
The biggest challenge at this stage is the inability to scale or rapidly deploy new models. Manual processes often lead to errors and inefficiencies, making it difficult for businesses to realize the full potential of machine learning.
MLOps Level 1: ML Pipeline Automation
The goal of MLOps level 1 is to introduce ML pipeline automation and enable continuous training (CT) of models. This level is ideal for businesses that need to keep models updated with fresh data while minimizing manual intervention.
Characteristics
- Automated Pipelines: Tasks like data preprocessing, model training, and validation are automated using MLOps solutions.
- Continuous Training: Models are automatically retrained using new data, ensuring they remain accurate as environments change.
- Unified Pipeline: The same ML pipeline used in development is also applied in production, ensuring operational consistency.
- Automated Deployment: New model versions are automatically deployed into production, minimizing manual work.
- Data and Model Validation: The pipeline validates incoming data and the model’s performance to ensure only high-quality models are deployed.
Challenges
While MLOps platforms at this level reduce manual work, businesses may still face MLOps challenges when trying to quickly implement new machine learning ideas or manage multiple pipelines. Continuous deployment (CD) for entirely new ML models may still require additional automation.
MLOps Level 2: CI/CD Pipeline Automation
At MLOps level 2, businesses implement a fully automated CI/CD pipeline that allows rapid deployment and retraining of models. This level is best suited for organizations that need to retrain models daily or even hourly, and where scalability is essential for operations.
Characteristics
- Source Control and Versioning: All code, model versions, and configurations are tracked using source control systems like Git, ensuring transparency.
- Automated CI/CD: New model versions are automatically integrated, tested, and deployed across multiple environments using a fully automated MLOps platform.
- Automated Retraining: If a model’s performance degrades, automated triggers initiate model retraining and redeployment.
- Full Monitoring and Feedback: MLOps software continuously monitors model performance and makes adjustments as needed.
- End-to-End Automation: From data ingestion to model deployment and monitoring, the entire lifecycle is fully automated, allowing the business to scale effortlessly.
Challenges
While MLOps as a service offers full automation at this level, it requires substantial investment in both infrastructure and expertise. This approach is typically used by tech-driven companies that need to retrain and redeploy models quickly across multiple servers.
Partner with a Trusted Digital Transformation Services Company
Implementing MLOps can be complex, which is why partnering with a trusted digital transformation services provider can make all the difference.
That’s where Matellio comes in!
With our tailored MLOps consulting services, we help businesses automate their ML pipelines, ensure seamless scalability, and achieve faster, more reliable model deployments. Whether you’re just starting out or need a fully automated CI/CD system, our team of experts can guide you through every step of your MLOps journey.
- Tailored Solutions: Customized MLOps strategies built to match your unique business needs.
- End-to-End Expertise: Full lifecycle support from pipeline setup to model monitoring.
- Fast Implementation: Accelerated deployment with automated CI/CD pipelines.
- Scalable Architecture: MLOps platforms designed to grow as your business expands.
- Seamless Integration: Easy integration with existing systems and cloud infrastructure.
- AI and ML Expertise: Proven experience in advanced AI and machine learning technologies.
- Secure and Compliant: Strong focus on data security, privacy, and regulatory compliance.
Partner with Matellio and Get a No-Obligation Quote for Your MLOps Project.
Conclusion
In today’s fast-paced, data-driven world, MLOps services are no longer a luxury but a necessity. Whether you are a small startup or a large enterprise, the ability to deploy, monitor, and scale machine learning models efficiently is crucial to staying competitive. By adopting MLOps solutions, you unlock new levels of automation, scalability, and collaboration across teams, ensuring your machine learning investments deliver real, measurable value.
At Matellio, we understand the challenges and opportunities that come with implementing MLOps. With our tailored MLOps consulting services, we help businesses streamline their machine learning workflows, enabling faster deployments, continuous integration, and robust model management. Whether you’re just starting with machine learning or need help with modern AI integration services, our team of experts will guide you through every step of the process.
Ready to supercharge your machine learning operations? Contact us today to start your MLOps journey!
FAQs
The cost depends on the complexity of your ML workflows, required features, and the scale of your operations. Contact us for a custom quote. Depending on the level of automation and your current infrastructure, setup times can range from a few weeks to several months. Not necessarily. With Matellio’s support, we provide ongoing management, monitoring, and optimization services as part of our MLOps consulting services. MLOps improves model deployment speed, ensures continuous monitoring, automates retraining, and enhances scalability—making your AI efforts more efficient and cost-effective. Absolutely. Our MLOps platforms are designed to integrate seamlessly with your existing systems, cloud infrastructure, and data pipelines. Q1. How much does it cost to implement MLOps services?
Q2. How long does it take to set up an MLOps pipeline?
Q3. Do I need an in-house team to manage MLOps after implementation?
Q4. What are the primary benefits of MLOps services for my business?
Q5. Can MLOps be integrated with our existing IT infrastructure?