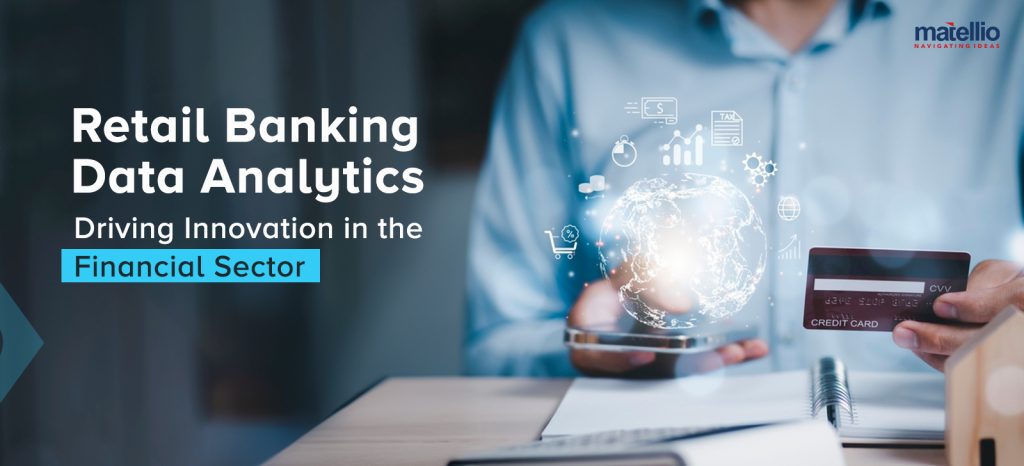
Data has become a cornerstone in the modern retail banking landscape. With the increasing digitization of financial services, retail banks are accumulating vast amounts of data from various channels, including customer transactions, social media interactions, and mobile banking activities. When harnessed effectively, this data can provide invaluable insights that drive better decision-making, improve customer experiences, and enhance operational efficiency.
Retail banking data analytics uses advanced data analysis techniques to extract actionable insights from these vast datasets. It is crucial in helping banks understand customer behavior, optimize their products and services, and stay competitive in an increasingly digital market. By leveraging data analytics, retail banks can personalize customer interactions, reduce risks, and streamline operations, ultimately leading to increased profitability and customer satisfaction.
Core Components of Retail Banking Data Analytics
Understanding the core components of retail banking data analytics is essential for any bank looking to leverage data effectively. Each component plays a unique role in providing comprehensive insights into different areas of the banking business:
Customer Data Analytics
This involves analyzing customer behavior, preferences, and demographics to better understand their needs. By segmenting customers based on their behavior, banks can offer personalized products and services to enhance customer satisfaction and loyalty.
Transaction Analytics
Analyzing transaction patterns allows banks to identify trends, detect anomalies, and prevent fraud. By monitoring transaction data, banks can gain insights into customer spending habits, which can be used to tailor financial products and services.
Risk Management Analytics
Risk management is critical in banking, and data analytics plays a significant role in assessing and mitigating risks. By analyzing credit scores, payment histories, and other relevant data, banks can make informed decisions on loan approvals and manage potential risks more effectively.
Product Performance Analytics
This retail banking data analytics component focuses on measuring the success of banking products and services. By analyzing sales data, customer feedback, and market trends, banks can identify which products are performing well and which need improvement.
Operational Analytics
Operational analytics involves optimizing internal processes to improve efficiency and reduce costs. By analyzing data from various operations, banks can identify bottlenecks, streamline workflows, and enhance overall operational efficiency.
Types of Data Analytics in Retail Banking
Retail banking data analytics can be categorized into several types, each serving a different purpose:
1. Descriptive Analytics
This type of analytics focuses on understanding what has happened in the past. It involves analyzing historical data to identify trends and patterns, providing insights into past performance.
2. Diagnostic Analytics
Diagnostic analytics goes a step further by exploring the reasons behind past performance. It helps banks understand why certain events occurred, enabling them to address issues and optimize future strategies.
3. Predictive Analytics
Predictive analytics uses historical data and ML algorithms to forecast future trends. In retail banking, this can be used to predict customer behavior, identify potential risks, and anticipate market changes.
4. Prescriptive Analytics
This type of analytics provides recommendations for actions based on data analysis. In retail banking, prescriptive analytics can suggest optimal pricing strategies, marketing campaigns, and risk management approaches.
5. Real-Time Analytics
Real-time analytics involves analyzing data as it is generated. It is particularly useful in detecting fraud, monitoring transactions, and providing instant insights for decision-making.
Benefits of Data Analytics in Retail Banking
Implementing data analytics in retail banking offers numerous benefits that can significantly enhance the bank’s performance and customer satisfaction:
Personalized Customer Experiences
Data analytics allows banks to understand their customers better and offer personalized products and services. By analyzing customer data, banks can tailor their offerings to meet individual needs, increasing customer satisfaction and loyalty.
Enhanced Risk Management
With data analytics, banks can assess and manage risks more effectively. Predictive analytics can help banks identify potential hazards before they become significant issues, enabling proactive risk management.
Increased Operational Efficiency
Banks can pinpoint inefficiencies and optimize processes by leveraging digital transformation services and analyzing data from various operations. This approach reduces costs enhances productivity, and streamlines overall operations, driving significant efficiency improvements.
Improved Decision-Making
Data-driven decision-making is more accurate and reliable. By using data analytics, banks can make informed decisions based on real-time insights, reducing the risk of errors and enhancing overall decision quality.
Better Marketing Strategies
Data analytics provides in-depth insights into customer behavior and preferences. It enables banks to create targeted marketing campaigns that improve the effectiveness of marketing efforts and maximize ROI.
Revenue Growth
By identifying high-performing products and services, banks can focus their resources on areas with the highest growth potential. Data analytics also helps identify new opportunities and optimize pricing strategies to increase revenue.
Regulatory Compliance
Data analytics in retail banking can assist banks in complying with regulatory requirements by monitoring transactions, detecting anomalies, and generating compliance reports. This reduces the risk of regulatory penalties and enhances the bank’s reputation.
Also Read: Retail Banking Analytics: How Data-Driven Insights Are Shaping the Future of Banking
Wish to Leverage Advanced Analytics for Driving Your Business Growth?
Fill Out the Form!
Implementing a Data Analytics Strategy in Retail Banking
Implementing a successful data analytics strategy in retail banking is a complex, multi-step process that requires careful planning and execution. Below is an elaboration of each step, highlighting how expert assistance can be invaluable at every stage of the implementation:
Defining Objectives and KPIs
The first step in implementing a data analytics strategy is to clearly define your objectives and establish key performance indicators (KPIs) that will measure the success of your initiatives. These objectives should align with your overall business goals and customer needs, ensuring the analytics efforts are focused and effective.
Our team can help you articulate clear, actionable objectives tailored to your specific banking environment. They will help you identify the most relevant KPIs that will not only measure success but also drive continuous improvement. Leveraging their industry experience, our experts can ensure that your objectives are realistic and aligned with your organization’s strategic vision.
Data Collection and Integration
Once your objectives are set, the next step is to gather data from various sources, including customer transactions, social media interactions, mobile banking activities, and more. The data must be integrated into a centralized platform for seamless analysis. This process often involves cleaning and standardizing the data to ensure accuracy and consistency, which is critical for reliable insights.
Our experts identify and integrate diverse data sources into a unified platform. They can assist in selecting the right tools for data analytics in retail banking and ensure that your data is clean and standardized to meet the highest quality standards. By leveraging advanced techniques and industry best practices, our team ensures that your data is accurate and ready for analysis, providing a solid foundation for all subsequent steps.
Choosing the Right Tools
The success of your data analytics strategy hinges on selecting the right tools that suit your specific banking needs. The tools should offer scalability, flexibility, and ease of use, enabling your team to handle the volume and complexity of your data effectively.
With extensive knowledge of the latest analytics tools and technologies, our experts can help you choose the most suitable solutions for your organization. They consider your current infrastructure, future growth plans, and specific data analytics requirements to recommend tools that provide the best fit. Our experts also assist in configuring these tools to maximize their efficiency and ensure seamless integration into your existing systems.
Building a Skilled Team
Building the right team is another significant step in implementing a successful retail banking data analytics strategy, but it comes with challenges. Assembling a team of data analysts, data scientists, and IT professionals from scratch can be time-consuming and costly. The process often involves recruiting, training, and upskilling staff, which can strain resources and delay the implementation of your analytics strategy.
You can trust our team of experts with extensive experience in the same field to avoid these hassles. Our experts are ready to hit the ground running, eliminating the need for lengthy training. By partnering with us, you can focus on your core business. At the same time, we ensure that your analytics strategy is executed with precision and efficiency, delivering the results you need without any unnecessary delays or additional costs.
Data Analysis and Interpretation
Once the tools and team are in place, the next step is to analyze the data and extract actionable insights. This involves interpreting the data to align with your business objectives and ensuring that the insights are communicated clearly to all relevant stakeholders.
Our team of experts specializes in translating complex data into actionable insights. They work closely with your team to ensure that the analysis is aligned with your objectives and that the insights are presented in a clear, concise manner that resonates with stakeholders at all levels. Our Data Consultation Services offer additional support, providing customized advice and strategies to help you make the most of your data insights.
Implementation and Optimization
Implementing the insights gained from retail banking data analytics into your banking operations is a critical step in the process. Continuous monitoring and optimization of your strategies based on feedback and new data are essential to ensure ongoing success. Regular reviews of your analytics strategy help maintain alignment with your business goals and adapt to changing market conditions.
Our experts assist in seamlessly integrating the insights into your banking operations, ensuring that the implementation is smooth and effective. They also provide ongoing support to monitor the results and optimize strategies as needed. With a focus on continuous improvement, our experts help you stay agile, adapting to new challenges and opportunities as they arise.
Data Governance and Security
Establishing robust data governance policies is vital to ensure data quality, security, and compliance with regulations. Protecting customer data through encryption, access controls, and regular audits is not just a regulatory requirement but also a critical aspect of maintaining customer trust.
Our experts bring a deep understanding of data governance and security best practices to your organization. They assist in developing and implementing comprehensive data governance frameworks that ensure data integrity and compliance with regulatory standards. Our team also provides guidance on implementing advanced security measures, safeguarding your customer data against breaches, and ensuring that your organization adheres to all relevant regulations.
Use Cases of Data Analytics in Retail Banking
Data analytics can be applied to various aspects of retail banking, providing valuable insights and improving overall performance. Here are some key use cases of data analytics:
Customer Segmentation and Personalization
Data analytics allows banks to segment their customers based on behavior, demographics, and preferences. This enables personalized marketing and product offerings, improving customer engagement and satisfaction.
Fraud Detection and Prevention
By analyzing transaction data in real time, banks can detect suspicious activities and prevent fraud. Data analytics in retail banking can also employ predictive models to identify potential fraud before it occurs, significantly reducing financial losses.
Customer Churn Prediction
Predictive analytics can identify customers who are likely to leave the bank, allowing for targeted retention strategies. By addressing the factors contributing to churn, banks can improve customer loyalty and reduce attrition rates.
Credit Scoring and Risk Assessment
Retail banking data analytics enhances credit scoring models by incorporating additional data points, such as social media behavior and transaction history. This leads to more accurate risk assessments and better-informed lending decisions.
Product Development and Optimization
Analyzing customer feedback and market trends helps banks develop new products and optimize existing ones. This ensures that the bank’s offerings meet customer needs and remain competitive in the market.
Regulatory Compliance and Reporting
Data analytics in retail banking simplifies the process of generating compliance reports by automating data collection and analysis. This ensures timely and accurate reporting, reducing the risk of regulatory penalties.
Challenges and Solutions in Retail Banking Data Analytics
Implementing data analytics in retail banking comes with its own set of challenges. Here are some common challenges and their solutions:
Data Privacy and Security
- Challenge: Protecting customer data while complying with regulations such as GDPR.
- Solution: Implement robust security measures, including encryption, access controls, and regular audits. Ensure compliance with data protection regulations through continuous monitoring and updates.
Integration with Legacy Systems
- Challenge: Difficulty in integrating new analytics tools with existing legacy systems.
- Solution: Use middleware solutions to bridge the gap between legacy systems and new technologies. Gradually phase out legacy systems and transition to modern, scalable platforms.
Data Quality and Accuracy
- Challenge: Ensuring the accuracy and consistency of data across multiple sources.
- Solution: Implement data governance policies that standardize data collection and management processes. Regularly audit and clean the data to maintain its quality and accuracy.
Talent Shortage
- Challenge: Lack of skilled data analysts and data scientists in the banking sector.
- Solution: Invest in training and development programs to build in-house expertise. Consider partnering with external analytics firms to access specialized skills.
Scalability
- Challenge: Managing the increasing volume of data as the bank grows.
- Solution: Adopt cloud-based analytics solutions that offer scalability and flexibility. Additionally, integrating cloud integration services ensures that your data architecture is designed to handle future growth, allowing for seamless expansion as your data needs evolve.
Cultural Resistance
- Challenge: Resistance to change from employees who are accustomed to traditional decision-making processes.
- Solution: Foster a data-driven culture by promoting the benefits of data analytics. Provide training and support to help employees transition to new analytics tools and processes.
Also Read: Harnessing the Power of Data Analytics in Banking: A Strategic Investment for Your Business
Beat These Challenges With The Expert Guidance!
Contact Us!
Future Trends in Retail Banking Data Analytics
The future of retail banking data analytics is set to be shaped by several key trends:
AI-Driven Predictive Analytics
The use of artificial intelligence (AI) to enhance predictive analytics is becoming increasingly prevalent. By leveraging AI integration services, AI-driven models can analyze vast amounts of data quickly, providing more accurate predictions and deeper insights.
Real-Time Data Processing
The demand for real-time analytics is growing as banks seek to make faster decisions and respond to customer needs immediately. Real-time data processing will become a standard feature in data analytics platforms.
Advanced Customer Segmentation
Banks will increasingly use data analytics to create highly targeted customer segments based on behavior, preferences, and demographics. This will enable more personalized and effective marketing strategies.
Cloud-Based Analytics
The adoption of cloud computing in data analytics will continue to rise, offering banks scalability, flexibility, and cost savings. Cloud-based solutions also facilitate collaboration and data sharing across departments.
Data-Driven Innovation
Banks will leverage data analytics to drive innovation in product development, customer service, and operational processes. Data-driven insights will enable banks to stay ahead of the competition and meet evolving customer needs.
RegTech Integration
Regulatory technology (RegTech) solutions will become more integrated with data analytics platforms, helping banks manage compliance more efficiently. This trend will be driven by the increasing complexity of regulatory requirements.
Enhanced Personalization
The trend towards hyper-personalization will continue, with banks using data analytics to tailor every aspect of the customer experience. This will include personalized product recommendations, communication strategies, and financial advice.
Also Read: Data Analytics in Banking and Finance: Shaping the Future of Financial Services
Want to Embrace The Future of Retail Banking With the Latest Trends?
Connect With Us Now!
How Can Matellio Help with Retail Banking Data Analytics?
Retail banking data analytics is crucial for enhancing decision-making, improving customer experiences, and driving operational efficiency in the highly competitive banking sector. Leveraging advanced analytics solutions can significantly elevate your bank’s ability to extract actionable insights from vast amounts of data, ultimately leading to better business outcomes.
Choosing Matellio can be beneficial:
- Our team develops advanced analytics models specifically designed to analyze critical metrics such as customer behavior, transaction patterns, and market trends. These models are customized to align with your bank’s goals and the unique challenges of the retail banking sector.
- We implement data analytics in retail banking to forecast customer behavior, market trends, and potential risks. This proactive approach allows you to optimize customer engagement strategies, improve risk management, and make informed decisions that enhance your bank’s competitive edge.
- We utilize cloud platforms to centralize access to analytics tools and insights, facilitating better collaboration among your team members. This integrated approach improves the efficiency and effectiveness of your data-driven strategies, ensuring seamless operations and enhanced customer experiences.
- Our technology consulting services guide you through the implementation and optimization of solutions for data analytics. We ensure that your team can fully leverage data insights to enhance decision-making, improve operational efficiency, and boost customer satisfaction.
You can fill out the form and reach out for our expert guidance to explore how you can optimize your retail banking operations with advanced data analytics solutions.
Q1. Can retail banking data analytics integrate with existing banking systems?
Yes, retail banking data analytics solutions can be seamlessly integrated with both legacy and modern banking systems. We design solutions that ensure a smooth integration process and continuity in your operations.
Q2. What are the costs associated with implementing retail banking data analytics?
Costs for implementing retail banking data analytics vary based on factors such as system complexity, technology requirements, and integration needs. We provide clear, detailed estimates tailored to your specific requirements and budget.
Q3. What support and maintenance do you offer for retail banking data analytics solutions?
We offer comprehensive support, including 24/7 assistance, regular system updates, performance optimization, and proactive monitoring. Our goal is to ensure that your retail banking data analytics system remains effective and up to date.
Q4. How is data migration handled during the implementation of retail banking data analytics?
Data migration is managed with a secure and structured approach, involving detailed planning and testing. We use backup and recovery solutions to ensure data integrity and minimize disruption during the transition.
Q5. How does Matellio ensure data security and compliance in retail banking data analytics solutions?
We implement robust security measures, including encryption, access controls, and regular audits, to ensure data protection and regulatory compliance. Our approach safeguards sensitive information and maintains the integrity of your retail banking data analytics system.