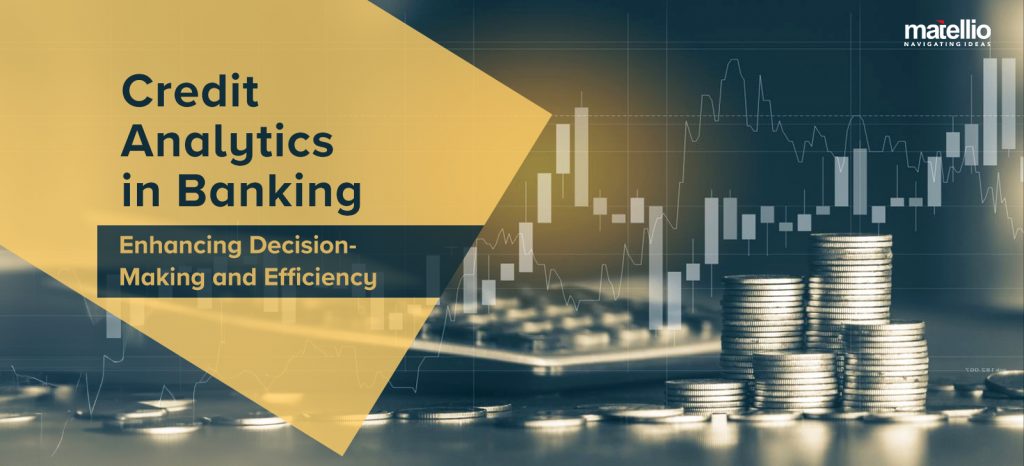
In traditional banking, assessing creditworthiness relies heavily on manual processes and limited data points, such as financial statements and credit histories. This method, while foundational, often lacked the precision needed for today’s dynamic financial environment. Enter credit analytics in banking, a transformative approach that leverages advanced data analysis techniques to enhance decision-making, risk management, and customer insights. By integrating vast amounts of data and employing sophisticated algorithms, credit analytics revolutionizes the way banks operate, offering a more accurate, efficient, and customer-centric approach to managing credit risk and opportunities.
Credit Analytics involves the systematic analysis of data to assess credit risk, predict customer behavior, and optimize financial decisions. It encompasses a wide range of methodologies and tools designed to analyze credit-related data, enabling banks to make informed decisions regarding lending, risk management, and customer relationship management. Data collection is the cornerstone of credit analytics. Effective data collection requires gathering data from various sources and ensuring its quality and relevance. To streamline data collection and ensure its accuracy, many banks utilize data consultation services, which help in identifying, acquiring, and managing the necessary data efficiently. Data analysis is crucial for transforming raw data into actionable insights. Several techniques are employed to analyze credit data: Effective reporting and visualization tools are essential for presenting the insights derived from data analysis in an understandable and actionable manner. Credit scoring models are fundamental tools in credit analytics, enabling banks to assess the creditworthiness of applicants. These models help in predicting the likelihood that a borrower will repay their debts, allowing banks to make informed lending decisions. These models primarily rely on a limited set of data points such as credit history, outstanding debt, and payment history. They provide a basic assessment of credit risk but may not capture the full financial behavior of an individual. These models incorporate a broader range of data, including behavioral data (such as spending habits and transaction patterns) and transactional data (such as frequency and types of transactions). This comprehensive approach offers a more nuanced and accurate assessment of credit risk. Assessing risk is a critical function of credit analytics, ensuring that banks can manage and mitigate potential losses effectively. These techniques help banks understand the risk profile of their credit portfolios and take proactive measures to manage those risks. This involves analyzing data specifically related to credit card usage to assess and manage the associated risks. It looks at factors such as spending patterns, repayment behaviors, credit limit utilization, and historical defaults to predict potential risks. Stress testing involves simulating extreme economic scenarios to evaluate the resilience of a bank’s credit portfolio. By assessing how the portfolio would perform under adverse conditions, banks can identify vulnerabilities and make necessary adjustments to their risk management strategies. Scenario analysis examines the impact of different hypothetical scenarios on credit risk. It allows banks to explore various “what-if” situations, such as changes in interest rates, economic downturns, or regulatory changes, to understand their potential impact on credit risk and prepare accordingly. Fraud detection is vital for protecting both the bank and its customers from financial losses due to fraudulent activities. By leveraging advanced analytics, banks can identify and prevent fraud more effectively. Specialized analytics tools are used to detect and prevent credit card fraud by identifying unusual patterns and behaviors. For example, a sudden spike in high-value transactions or transactions in different geographical locations within a short period may indicate fraudulent activity. Anomaly detection involves identifying deviations from normal behavior that may indicate fraud. For instance, if a customer typically makes small, regular purchases but suddenly starts making large, irregular purchases, this could be flagged as a potential anomaly. Behavioral analytics examines customer behavior to identify potential fraud risks. By analyzing patterns such as spending habits, transaction times, and purchase locations, banks can detect suspicious activities that deviate from the norm. Transform Your Credit Analytics Strategy with Our Experts! Contact Us! Credit analytics offers numerous benefits to banks, enhancing their operational efficiency and effectiveness in several key areas: Credit analytics in banking helps to identify high-risk customers at an early stage by analyzing various data points, including credit histories, transaction patterns, and behavioral indicators. This early identification allows banks to take preemptive measures, such as adjusting credit limits or offering tailored repayment plans, to mitigate potential risks. With advanced data analysis techniques, banks can perform more accurate risk assessments. By considering a wider range of data, including non-traditional data sources, banks can make more informed lending decisions. This reduces the likelihood of defaults and improves the overall health of the loan portfolio. Credit card data analytics provides banks with a deeper understanding of their customers’ financial behaviors and needs. By analyzing transactional data and spending patterns, banks can gain insights into customer preferences and financial habits. This understanding helps segment customers more effectively and identify opportunities for cross-selling and upselling. Armed with detailed customer insights, banks can offer personalized financial products and services that meet the specific needs of individual customers. Personalized offerings, such as tailored loan terms, customized credit limits, and targeted marketing campaigns, enhance customer satisfaction and loyalty. Credit analytics allows for the automation of various credit assessment processes. Automated systems can quickly analyze vast amounts of data, perform credit scoring, and make lending decisions without human intervention. Leveraging AI integration services speeds up the loan approval process and ensures consistency and reduces the potential for human error. Automation and advanced analytics reduce the need for manual data processing and analysis. This minimizes the chances of errors that can occur during manual handling of data, leading to more accurate and reliable credit assessments. Credit analytics in banking helps comply with regulatory requirements by providing transparent and well-documented credit assessment processes. By maintaining comprehensive records and audit trails, banks can demonstrate their adherence to regulations and avoid potential fines or penalties. Advanced credit analytics solutions generate detailed reports and documentation for each credit decision. This transparency ensures that all decisions can be audited and reviewed, providing clear justifications for lending decisions and ensuring regulatory compliance. Credit analytics enables banks to develop better diversification strategies for their loan portfolios. By analyzing the risk profiles of different customer segments and industries, banks can allocate resources more effectively and balance risk across their portfolios. Credit analytics plays a crucial role in detecting and preventing fraud. By analyzing transaction patterns and identifying anomalies, banks can detect fraudulent activities at an early stage. This includes leveraging credit card fraud analytics to specifically monitor credit card transactions for unusual patterns and behaviors. This enables them to take immediate action to prevent further fraudulent transactions and mitigate losses. Enhanced fraud detection mechanisms provide increased security for both the bank and its customers. By protecting customer accounts from fraudulent activities, banks can build trust and gain confidence among their customers, ultimately enhancing customer loyalty and satisfaction. Want To Develop Credit Analytics Solution for Maximum ROI? Connect With Us! Credit analytics in banking can help various operations improve efficiency and outcomes. Here are some detailed use cases: Credit analytics enables the automation of the loan origination and approval process. Advanced algorithms analyze an applicant’s credit history, income, employment status, and other relevant data points to make quick and accurate lending decisions. This integration of digital transformation services reduces the time required for loan processing and improves customer satisfaction. By incorporating a wider range of data sources, including transactional and behavioral data, credit analytics provides a more comprehensive assessment of an applicant’s creditworthiness. This leads to more accurate evaluations and reduces the risk of approving loans to high-risk individuals. Credit analytics in banking allows us to monitor customer behavior continuously and adjust credit limits dynamically. For example, if a customer demonstrates responsible credit usage and timely repayments, their credit limit can be increased. Conversely, if there are signs of financial distress, the credit limit can be decreased to mitigate risk. Banks can use credit analytics to segment their customer base into different risk profiles and behavioral groups. This segmentation enables targeted marketing campaigns that cater to the specific needs and preferences of each group. For instance, high-value customers can be offered premium products, while high-risk customers might receive tailored financial advice and products designed to improve their financial health. Credit analytics helps banks develop more effective debt collection strategies by analyzing customer risk profiles and behavior. Customers who are likely to repay with a reminder can be targeted with softer collection tactics, while those who pose a higher risk of default can be approached with more assertive measures. This tailored approach increases the efficiency of debt collection and reduces recovery costs. Credit analytics ensures that banks can comply with regulatory standards by providing accurate and timely reporting. Automated systems generate detailed reports that meet regulatory requirements, reducing the risk of non-compliance and associated penalties. This transparency also enhances the bank’s credibility with regulators and stakeholders. Credit card risk analytics enables banks to optimize their credit portfolios by balancing risk and return. By continuously monitoring portfolio performance and assessing the risk associated with different segments, banks can make informed decisions about where to allocate resources. This strategic approach maximizes returns while maintaining an acceptable level of risk. Discover How to Boost Your Bank’s Profitability with Credit Analytics! Fill The Form! The future of credit analytics in banking is shaped by advancing technology and evolving industry practices. Here are some detailed future trends: Continued improvement in AI and ML algorithms will enhance the accuracy and efficiency of credit analytics. These advancements will enable more precise risk assessments after identifying patterns and correlations that may not be apparent through traditional methods. Machine learning models will continuously learn and adapt to new data, improving their predictive capabilities over time. AI-driven credit analytics solutions will allow for the creation of highly personalized credit products tailored to individual customer profiles. By analyzing vast amounts of data, banks can offer customized loan terms, interest rates, and credit limits that match the specific needs and behaviors of each customer. Leveraging large and diverse datasets will enable more comprehensive and nuanced credit assessments. By incorporating non-traditional data sources such as utility payments, and mobile phone usage, banks can gain a fuller understanding of a customer’s financial behavior and creditworthiness. The utilization of big data will enhance predictive insights, allowing banks to anticipate customer needs and market trends more effectively. This proactive approach will enable banks to develop innovative products and services that meet emerging demands and mitigate potential risks. Real-time data processing and analytics will allow for more timely and dynamic credit decision-making. By analyzing live data streams, banks can make instant lending decisions, adjust credit limits on the fly, and respond quickly to changes in a customer’s financial situation. This ability will improve customer experience and satisfaction. Real-time analytics can help in detecting and preventing fraud. By continuously monitoring transactions and identifying anomalies as they occur, banks can take immediate action to prevent fraudulent activities. Developments in regulatory technology (RegTech) will provide better tools for compliance and risk management. Automated compliance systems will streamline the process of adhering to regulatory requirements, reducing the burden on bank staff and minimizing the risk of non-compliance. RegTech solutions will enable real-time regulatory reporting, ensuring that banks can meet their compliance obligations promptly and accurately. This transparency will enhance the relationship between banks and regulators, fostering a more robust financial ecosystem. The adoption of cloud computing will offer banks greater scalability and flexibility in their credit analytics operations. Cloud-based solutions can handle vast amounts of data and complex analytical processes. It can help banks scaling the operations up or down based on demand. This flexibility, facilitated by cloud integration services, will enable banks to innovate and adapt quickly to changing market conditions. The costs associated with maintaining and upgrading the on-premises infrastructure can be reduced. By leveraging cloud services, banks can lower their IT expenses while benefiting from the latest advancements in data storage, processing, and security. Cloud-based platforms will facilitate better collaboration and integration across different departments and systems within a bank. This seamless connectivity will enable more efficient data sharing and analytics, leading to improved decision-making and operational efficiency. Credit analytics in banking is vital for assessing creditworthiness, managing risk, and enhancing customer satisfaction. Implementing advanced credit analytics solutions can significantly improve your bank’s ability to make informed lending decisions and optimize credit portfolios. Here are some key areas where choosing us can be beneficial: You can fill out the form and reach out for our expert’s guidance to explore how you can upgrade your banking operations with credit analytics solutions. Yes, credit analytics systems can be seamlessly integrated with both legacy and modern banking infrastructure. We design hybrid solutions that work with your current IT setup to ensure a smooth transition and continuity in your operations. Costs for implementing credit analytics vary based on factors such as system complexity, technology requirements, and integration needs. We provide clear, detailed estimates tailored to your specific requirements and budget. We offer comprehensive support, including 24/7 assistance, regular system updates, performance optimization, and proactive monitoring. Our goal is to ensure that your credit analytics system remains effective and up to date. Data migration is managed with a secure and structured approach, involving detailed planning and testing. We use backup and recovery solutions to ensure data integrity and minimize disruption during the transition. We implement robust security measures, including encryption, access controls, and regular audits, to ensure data protection and regulatory compliance. Our approach safeguards sensitive information and maintains the integrity of your credit analytics system. What is Credit Analytic?
Key Components of Credit Analytics in Banking
Data Collection
Sources of Data (Internal and External)
Types of Data
Data Analysis Techniques
Statistical Analysis
Predictive Modeling
Machine Learning and AI
Reporting and Visualization
Dashboards and Reporting Tools
Methodologies Used for Credit Analytics in Banking
Credit Scoring Models
Traditional vs. Modern Scoring Models
Traditional Models
Modern Models
Risk Assessment Techniques
Credit Card Risk Analytics
Stress Testing
Scenario Analysis
Fraud Detection
Credit Card Fraud Analytics
Anomaly Detection
Behavioral Analytics
Benefits of Credit Analytics in Banking
Improved Risk Management
Early Identification of High-Risk Customers
More Accurate Risk Assessments Leading to Better Decision-Making
Enhanced Customer Insights
Deeper Understanding of Customer Needs and Behaviors
Ability to Offer Personalized Products and Services
Increased Efficiency
Automation of Credit Assessment Processes
Reduced Manual Effort and Errors
Better Regulatory Compliance
Ensuring Adherence to Regulatory Requirements
Transparent and Auditable Decision-Making Processes
Optimized Portfolio Management
Better Diversification Strategies
Fraud Reduction
Early Detection and Prevention of Fraudulent Activities
Increased Security for Both the Bank and Its Customers
Use Cases of Credit Analytics in Banking
Loan Origination and Approval
Automating the Decision-Making Process
Enhancing the Accuracy
Credit Limit Management
Dynamically Adjusting Credit Limits
Customer Segmentation
Grouping Customers
Debt Collection
Optimizing Collection Strategies
Regulatory Reporting
Ensuring Compliance with Regulatory Standards
Portfolio Optimization
Balancing Risk and Return
Future Trends of Credit Analytics in Banking
AI and Machine Learning Advancements
Enhanced Accuracy and Efficiency
Personalized Credit Products
Big Data Utilization
Comprehensive Credit Assessments
Predictive Insights
Real-Time Analytics
Timely and Dynamic Decision-Making
Fraud Detection and Prevention
RegTech Innovations
Enhanced Compliance Tools
Real-Time Regulatory Reporting
Cloud Computing
Scalability and Flexibility
Cost Efficiency
Enhanced Collaboration and Integration
How Can Matellio Help with Credit Analytics in Banking?
Q1. Can credit analytics integrate with existing systems?
Q2. What are the costs associated with implementing credit analytics?
Q3. What support and maintenance do you offer for credit analytics?
Q4. How is data migration handled during the implementation of credit analytics?
Q5. How does Matellio ensure data security and compliance in credit analytics solutions?